Terceira-S05-S2 Machine Learning in Regional Science: Perspectives, Methods, and Applications
Tracks
Special Session
Thursday, August 29, 2024 |
9:00 - 10:30 |
S03 |
Details
Chair: Kevin Credit, National Centre for Geocomputation, Maynooth, Ireland; Katarzyna Kopczewska, University of Warsaw, Poland
Speaker
Dr. R. Daniel Jonsson
Senior Researcher
KTH Royal Institute Of Technology
Are generative models for synthetic populations enough?
Author(s) - Presenters are indicated with (p)
R. Daniel Jonsson (p)
Discussant for this paper
Korneliusz Pylak
Abstract
In this paper we discuss how both established methods and new machine learning approaches to the process of creating synthetic populations solve part of the problem very well but fails to address a crucial policy relevant part. We briefly outline the state of the art and touch on some recent research of our own to establish what existing methods do well. In the extended abstract we then argue that these methods is less help than we might think when it comes to analysing counterfactual och future scenarios. A forthcoming paper adds examples from computer simulations to establish this. This conference contribution can be viewed as a call for future research into the intersection of population synthesis, land-use and transport interaction, and planning decision support.
Dr. Gianluca Monturano
Ph.D. Student
Università di Modena e Reggio Emilia - Dipartimento di Economia Marco Biagi
Anticipating Delays in Cohesion Infrastruture Projects by Machine Learning
Author(s) - Presenters are indicated with (p)
Giuseppe Coco, Gianluca Monturano (p), Giuliano Resce
Discussant for this paper
R. Daniel Jonsson
Abstract
Regional fragilities in incorporating the benefits of cohesion policies are partially due to difficulties in allocating the resources programmed. The efficiency of the allocation mechanism depends on several factors: project-related features, territorial characteristics, and institutional features such as coordination among public authorities. This paper proposes a machine learning model for predicting lags in cohesion project execution. Lags in policymaking are measured on cohesion projects monitored by opencoesione.gov.it. We measure execution times in different phases: (i) planning, (ii) execution, and (iii) conclusion. Results show that potential lags can be predicted and that institutional factors matter.
Dr. Korneliusz Pylak
Post-Doc Researcher
Lublin University of Technology
Textual Alchemy: Predicting Company Innovation by Deciphering Unstructured Website Content in Time and Space
Author(s) - Presenters are indicated with (p)
Korneliusz Pylak (p)
Discussant for this paper
Gianluca Monturano
Abstract
Our study pioneers a holistic approach, offering early indicators of upcoming innovations and deepening our understanding of the complex connections within the geography of innovation by decoding unstructured website text. In the rapidly changing landscape of innovation research, traditional methodologies based on well-established secondary data sources are being extended with cutting-edge approaches. This paper uncovers the dynamics of innovation in companies by exploiting a massive body of unstructured textual website data. Moving away from conventional static analyses of company websites, we use advanced web scraping, social network analysis and natural language processing techniques to introduce a temporal dimension to our exploration. Focusing on the Polish business landscape, our study uses WebArchive's database of websites, which includes more than ten thousand corporate entities that filed patents between 2001 and 2023.
Our methodology geolocates each company in detail, embedding it in a well-described socio-economic context that takes into account organisational structure, economic and technological diversity and local knowledge complexity. In the study, we use patent data extracted from extensive databases and a nuanced exploration of textual representations of innovations. To uncover patterns and insights from unstructured text, we use advanced topic modelling tools such as Latent Dirichlet Allocation (LDA), Latent Semantic Analysis (LSA), Correlated Topics Models (CTM) and word embeddings such as GloVe. Furthermore, transformational natural language processing (NLP) capabilities, including state-of-the-art Transformer models, were included in our analysis.
This innovative approach goes beyond traditional boundaries, predicting a company's innovation based on changes in the textual content of its website over time. A temporal perspective allows us to capture the evolution of innovative activities, providing a holistic understanding of the innovation process within a single company. At the same time, we address the spatial dimension, considering the geographical proximity of innovative actors to capture the interplay between them. This detailed exploration sheds light on the geography of knowledge production and relationships, revealing how spatial dynamics shape innovation in the Polish business landscape.
Our methodology geolocates each company in detail, embedding it in a well-described socio-economic context that takes into account organisational structure, economic and technological diversity and local knowledge complexity. In the study, we use patent data extracted from extensive databases and a nuanced exploration of textual representations of innovations. To uncover patterns and insights from unstructured text, we use advanced topic modelling tools such as Latent Dirichlet Allocation (LDA), Latent Semantic Analysis (LSA), Correlated Topics Models (CTM) and word embeddings such as GloVe. Furthermore, transformational natural language processing (NLP) capabilities, including state-of-the-art Transformer models, were included in our analysis.
This innovative approach goes beyond traditional boundaries, predicting a company's innovation based on changes in the textual content of its website over time. A temporal perspective allows us to capture the evolution of innovative activities, providing a holistic understanding of the innovation process within a single company. At the same time, we address the spatial dimension, considering the geographical proximity of innovative actors to capture the interplay between them. This detailed exploration sheds light on the geography of knowledge production and relationships, revealing how spatial dynamics shape innovation in the Polish business landscape.
Mr Ali Sobhani
Ph.D. Student
Utrecht University
Media, perception and location behaviour: crime reporting and house prices in U.S. cities
Author(s) - Presenters are indicated with (p)
Ali Sobhani (p), Evert Meijers, Rodrigo Cardoso, Martijn Burger
Abstract
Cities have been seen by their residents not only through their daily life experiences but also by means of a wide range of media resources that create an image in their minds. How this image can shape the behavioural decisions of people regarding cities and the places they live has rarely been studied. Employing new techniques and technologies in Natural Language Processing (NLP), and focusing on the impact of the perception of crime as conveyed by media on the typical housing prices in US cities, this paper tries to investigate if media representations of cities can have a spatial impact at large geographical scales. The paper puts forward novel techniques of application of NLP to urban studies and proposes a pipeline of different NLP techniques to study news corpuses about cities. The results show that the image of cities in the media regarding crime has a significant correlation with typical housing prices in US cities.
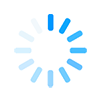