Terceira-G31-O3 Methods in Regional Science or Urban Analysis
Tracks
Ordinary/Refereed
Friday, August 30, 2024 |
9:00 - 10:30 |
S13 |
Details
Chair: José-Miguel Rebolho
Speaker
Dr. Aneta Chmielewska
Assistant Professor
Uniwersytet Warmińsko-Mazurski w Olsztynie
Real estate industry sustainable solutions (ESG) significance assessment - AI-powered algorithm implementation
Author(s) - Presenters are indicated with (p)
Marek Walacik (p), Aneta Chmielewska (p)
Discussant for this paper
José-Miguel Rebolho
Abstract
As the global imperative for sustainable development intensifies, the real estate industry stands at the intersection of environmental responsibility and economic viability. This research presents a comprehensive exploration of the significance of sustainable solutions within the real estate sector, employing advanced artificial intelligence (AI) algorithms to assess their impact. The study focuses on the integration of AI-powered tools in decision-making process analysis. The research meth-odology involves the development and implementation of AI algorithms capable of analyzing vast datasets related to real estate attributes. By leveraging machine learning techniques, the Al-gorithm assesses the significance of energy efficiency solutions along with other intrinsic and extrinsic attributes. The research examines the effectiveness of these solutions in relation to the influence on property prices with a framework based on an AI-driven algorithm. The findings aim to inform real estate professionals and investors about the tangible advantages of integrating AI technologies into sustainable solutions, promoting a more informed and responsible approach to industry practices. This research contributes to the growing interest in the connection of the real estate sector, sustainability, and AI, offering insights that can guide strategic decision-making. By implementing the random forest method in the real estate features significance assessment original methodology it has been shown that AI-powered algorithms can be a useful tool from the perspective of real estate price prediction. The methodology’s ability to handle non-linear relationships and provide insights into feature importance proved advantageous in comparison to the multiple regression analysis.
Mr José-Miguel Rebolho
Ph.D. Student
University of Coimbra, CeBER, Faculty of Economics
Updating IO models – A step forward for PReMMIA
Author(s) - Presenters are indicated with (p)
José-Miguel Rebolho (p), Luís Cruz, João-Pedro Ferreira
Discussant for this paper
Aneta Chmielewska
Abstract
Recent economic and societal challenges have highlighted the need to develop better estimates that specify, in a globalised world, the dynamics between trade, sectoral linkages, and transportation networks. Value chains become a topic that deserves more attention from the scientific community, the media, and those involved in international policy, but practitioners still struggle to access official data that allows them to update their modelling accuracy in an increasingly unpredictable world. In Portugal, as in most of the countries, Input-Output (I-O) data can be released with significant time lags, typically around five years, requiring the use of updating estimation techniques.
Nevertheless, it is possible to take advantage of additional statistical information dispersed across various sources to develop and update the sectoral linkages of an I-O model for the following years. These include Tables by Industry, National Accounts, Agriculture Census, National Forest Inventory, as well as, e.g., data on energy and electricity production. Our assumption is that the adequate integration of this information raises accuracy in the process of updating I-O tables, ensuring a more precise representation of the economic structure and, therefore, a better estimation of the multipliers.
In this paper, we aim to find the best method to regularly update the Portuguese Matrix of Technical Coefficients and Matrix of Leontief. The goal is to create an accurate model of the economic structure, using dispersed information from more recent years than the ones that are reported in national accounts. To estimate the core of these matrices in subsequent years, we will use bi-proportional matrix balancing algorithms, particularly RAS, Generalised RAS, and Sign Preserving Squared Differences. We will also assess potential errors by comparing the results produced by each method and official data from Portuguese National Accounts for previous years. Measures such as Mean Absolute Deviation and Weighted Absolute Difference will be employed to evaluate the disparity between the estimated core matrix and the actual data. Given the results and departing from the last year available (2017), we will estimate a new national I-O model for Portugal in 2023.
The following step will involve extending this approach to update PReMMIA (Portuguese Regions Model for Multi Impact Analysis), a Multi-Regional I-O (MRIO) model for Portugal. This updating procedure and corresponding matrices will enhance policymakers' capacity for both ex-ante and ex-post impact assessments affecting the country's regions, thus aiding informed decision-making at national and regional levels.
Nevertheless, it is possible to take advantage of additional statistical information dispersed across various sources to develop and update the sectoral linkages of an I-O model for the following years. These include Tables by Industry, National Accounts, Agriculture Census, National Forest Inventory, as well as, e.g., data on energy and electricity production. Our assumption is that the adequate integration of this information raises accuracy in the process of updating I-O tables, ensuring a more precise representation of the economic structure and, therefore, a better estimation of the multipliers.
In this paper, we aim to find the best method to regularly update the Portuguese Matrix of Technical Coefficients and Matrix of Leontief. The goal is to create an accurate model of the economic structure, using dispersed information from more recent years than the ones that are reported in national accounts. To estimate the core of these matrices in subsequent years, we will use bi-proportional matrix balancing algorithms, particularly RAS, Generalised RAS, and Sign Preserving Squared Differences. We will also assess potential errors by comparing the results produced by each method and official data from Portuguese National Accounts for previous years. Measures such as Mean Absolute Deviation and Weighted Absolute Difference will be employed to evaluate the disparity between the estimated core matrix and the actual data. Given the results and departing from the last year available (2017), we will estimate a new national I-O model for Portugal in 2023.
The following step will involve extending this approach to update PReMMIA (Portuguese Regions Model for Multi Impact Analysis), a Multi-Regional I-O (MRIO) model for Portugal. This updating procedure and corresponding matrices will enhance policymakers' capacity for both ex-ante and ex-post impact assessments affecting the country's regions, thus aiding informed decision-making at national and regional levels.
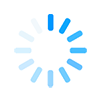