Terceira-S06-S2 RSPP Special Issue: A New Toolbox for Novel Research in Regional, Urban and Spatial Studies
Tracks
Special Session
Friday, August 30, 2024 |
9:00 - 10:30 |
S01 |
Details
Chair: Katarzyna Kopczewska, University of Warsaw, Poland
Speaker
Mr Federico Ninivaggi
Post-Doc Researcher
Università Di Macerata
Liveable Urban Spaces in Polycentric Territories: A Case Study for Central Italy
Author(s) - Presenters are indicated with (p)
Federico Ninivaggi (p), Mrs Eleonora Cutrini
Discussant for this paper
Alessio Bumbea
Abstract
In recent years, major cities have increasingly promoted urban policies to achieve a more sustainable environment, especially after the COVID-19 pandemic. Indeed, during lockdowns, with the forced restrictions on people’s mobility, spending more time at home has highlighted the centrality of the provision of neighborhood services. In this manner, COVID-19 reopened and boosted the debate on how to radically rethink urban spaces, in metropolitan areas but also in middle cities and polycentric territories. After Paris, with its mayor Hidalgo that launched the slogan of "city 15 minutes away", redefining urban strategies for resilience was also taken up by several Italian cities, including Milan, Rome and Bologna.
The notion of Carlos Moreno’s 15-minute city (Moreno et al., 2021) refers to an area where citizens can easily reach essential services through a 15 minutes’ walk. The 15-minute city is an urban planning concept focused on groups of people who have historically been excluded from planning, such as women, children, people with disabilities, and the elderly. Access to schools, green spaces, basic social infrastructures, and complementary activities for residents are considered essential to improve the well-being of local communities.
The ongoing structural funds programming and the National Recovery and Resilience Plan represent a unique opportunity for a sustainable redefinition of the urban development model. However, to be successful, public policies and regional planning need to be accompanied by result-oriented monitoring, reporting and evaluation processes. Transparent and spatially detailed information is extremely important to support collective decision-making and to develop evidence-based policies. In this respect, open digital navigation platforms, such as Google Maps and OpenStreetMap (OSM), are becoming increasingly popular among academics and practitioners as a precious source of knowledge, especially when official statistics are difficult or expensive to produce at a detailed spatial granularity.
Against this backdrop, this work aims to construct metrics able to evaluate progress in terms of accessibility to services related to basic needs and amenities, with a focus on mobility. Our case study focuses on the Marche region in Central Italy which is an emblematic example of the polycentric system of towns, rural areas and municipalities characterizing most of the Italian territory.
Based on our results, implications for policy and planning will be discussed. Moreover, we will provide possible avenues for future research and explain how the approach for a proximity metric based on OSM input categories is scalable, i.e., transferable across spatial scales without any significant adjustment.
The notion of Carlos Moreno’s 15-minute city (Moreno et al., 2021) refers to an area where citizens can easily reach essential services through a 15 minutes’ walk. The 15-minute city is an urban planning concept focused on groups of people who have historically been excluded from planning, such as women, children, people with disabilities, and the elderly. Access to schools, green spaces, basic social infrastructures, and complementary activities for residents are considered essential to improve the well-being of local communities.
The ongoing structural funds programming and the National Recovery and Resilience Plan represent a unique opportunity for a sustainable redefinition of the urban development model. However, to be successful, public policies and regional planning need to be accompanied by result-oriented monitoring, reporting and evaluation processes. Transparent and spatially detailed information is extremely important to support collective decision-making and to develop evidence-based policies. In this respect, open digital navigation platforms, such as Google Maps and OpenStreetMap (OSM), are becoming increasingly popular among academics and practitioners as a precious source of knowledge, especially when official statistics are difficult or expensive to produce at a detailed spatial granularity.
Against this backdrop, this work aims to construct metrics able to evaluate progress in terms of accessibility to services related to basic needs and amenities, with a focus on mobility. Our case study focuses on the Marche region in Central Italy which is an emblematic example of the polycentric system of towns, rural areas and municipalities characterizing most of the Italian territory.
Based on our results, implications for policy and planning will be discussed. Moreover, we will provide possible avenues for future research and explain how the approach for a proximity metric based on OSM input categories is scalable, i.e., transferable across spatial scales without any significant adjustment.
Dr. Sofia Wixe
Associate Professor
Jönköping International Business School
Which path do you choose, and does it matter? Industry trajectories and subsequent earnings of forced migrants
Author(s) - Presenters are indicated with (p)
Sofia Wixe (p), Johan Klaesson
Discussant for this paper
Federico Ninivaggi
Abstract
This paper uses a novel two-step approach to identify long-term consequences of earlier industry experience on later income. First, using sequence analysis, we identify typical industry trajectories that individuals follow during 1992-2011. Second, we estimate wage regressions for 2012-2021, where the assigned trajectory is the variable of interest, controlling for observed and unobserved individual characteristics as well as location. Our identification draws on a refugee placement policy of the early 1990s when our study population, forced migrants from the former Yugoslavian Republic, arrived in Sweden. The findings show that industry trajectories exhibit considerable stability over time and the only industry identified as a steppingstone into other industries is manufacturing. Migrants with inactive paths face long-term consequences in terms of receiving a lower income in future employment. Individuals entering knowledge-intensive services on the other hand reap higher incomes even if transferring to lower-wage industries. Overall, the results show strong persistence from earlier industry experiences.
Ms Céline Van Migerode
Ph.D. Student
KU Leuven
A spatially-explicit sensitivity analysis of urban definitions: uncovering implicit assumptions in the Degree of Urbanisation
Author(s) - Presenters are indicated with (p)
Céline Van Migerode (p), Ate Poorthuis, Ben Derudder
Discussant for this paper
Sofia Wixe
Abstract
There is no broadly shared agreement on how to define a city, neither in terms of methods nor in terms of thresholds or criteria to be used in these definitions. This can have profound implications: different delineation approaches lead to varying city boundaries, which in turn affect the quantitative analysis of, for example, economic development. In addition, deciding on delineation criteria inevitably involves making explicit and implicit assumptions about the fundamental nature of ‘the urban’. These assumptions may – unwittingly – reproduce urban realities experienced and observed by those expressing them, potentially leading to spatially unequally distributed implications. It is key to understand how specific criterion choices shape our understanding of ‘the urban’ and assess how, why and – especially – where a definition leads to specific sensitivities.
Against this backdrop, we introduce a methodological framework to conduct a spatially-explicit sensitivity analysis that aims to quantify the sensitivity of a location’s ‘urban’ classification across various criterion settings. Our methodological framework is spatially-explicit in the sense that it does not rely on aggregate statistics such as the urban population or number of cities per region, but instead focuses on the sensitivity of the classification of individual spatial units at the finest possible resolution. The final sensitivity map serves as a starting point for an in-depth examination and guides researchers to potentially interesting regions. By zooming in on these regions researchers can assess the link between the sensitivity and the underlying urbanisation pattern. In that way, they can gather a deeper understanding of the implications of certain criterion choices for specific types of urbanisation, and potentially uncover implicit assumptions tied to a delineation approach.
As empirical example, we apply the framework to the definition of the Degree of Urbanisation proposed by Dijkstra et al. (2021). Our initial results demonstrate that sensitivity is not equally distributed across the world. Certain regions (e.g. area around Dallas – Fort Worth) and specific types of urbanisation (e.g. desakota regions) exhibit higher sensitivity than others, pointing to potential skewness in the urban delineations of DEGURBA. This serves as an example to illustrate that, because there is no generally accepted ‘true’ definition of urbanisation, every urban definition inevitably requires making assumptions about the outlook of ‘the urban’. However, it is crucial to be aware of unequally distributed sensitivities when employing a specific definition. Our proposed methodological framework facilitates making the implications of sensitivities legible in a spatially-explicit manner.
Against this backdrop, we introduce a methodological framework to conduct a spatially-explicit sensitivity analysis that aims to quantify the sensitivity of a location’s ‘urban’ classification across various criterion settings. Our methodological framework is spatially-explicit in the sense that it does not rely on aggregate statistics such as the urban population or number of cities per region, but instead focuses on the sensitivity of the classification of individual spatial units at the finest possible resolution. The final sensitivity map serves as a starting point for an in-depth examination and guides researchers to potentially interesting regions. By zooming in on these regions researchers can assess the link between the sensitivity and the underlying urbanisation pattern. In that way, they can gather a deeper understanding of the implications of certain criterion choices for specific types of urbanisation, and potentially uncover implicit assumptions tied to a delineation approach.
As empirical example, we apply the framework to the definition of the Degree of Urbanisation proposed by Dijkstra et al. (2021). Our initial results demonstrate that sensitivity is not equally distributed across the world. Certain regions (e.g. area around Dallas – Fort Worth) and specific types of urbanisation (e.g. desakota regions) exhibit higher sensitivity than others, pointing to potential skewness in the urban delineations of DEGURBA. This serves as an example to illustrate that, because there is no generally accepted ‘true’ definition of urbanisation, every urban definition inevitably requires making assumptions about the outlook of ‘the urban’. However, it is crucial to be aware of unequally distributed sensitivities when employing a specific definition. Our proposed methodological framework facilitates making the implications of sensitivities legible in a spatially-explicit manner.
Mr Alessio Bumbea
Ph.D. Student
Universitas Mercatorum
Spatial bootstrapping using deep clustering methods: spatial machine learning applied to Lombardy high-tech businesses
Author(s) - Presenters are indicated with (p)
Alessio Bumbea (p), Giuseppe Espa, Andrea Mazzitelli
Discussant for this paper
Céline Van Migerode
Abstract
Our work introduces a novel spatial machine-learning approach based on Deep learing to analyse high-tech firms in Lombardy, Italy. We used the Deep Embedded Clustering (DEC) algorithm, which leverages an Autoencoder Neural Network to create meaningful strata of similar businesses. This notion of similarity is not given by an ex ante assumption of distance between the characteristics of businesses, but rather it is iteratively learned by the machine learning algorithm during training, by looking at the characteristics of the probability distribution of the clusters.
Our model considers the characteristics of businesses like their latitude and longitude, therefore it takes into account the geographical distance between the firms but it also takes into account several other characteristics like their economical performances and their sector of activity. This feature is essential to our analysis since it allowed us to create clusters that effectively mitigated the issues brought about by geographical inhomogeneity.
The DEC algorithm's role is crucial as it efficiently segments high-tech firms into coherent groups based on factors like sector activity and economic performance. This segmentation is not merely based on geographical proximity but also deeper, often hidden, interactions of business characteristics. Such a nuanced approach to clustering provides a more accurate representation of the high-tech business environment in Lombardy. This sophisticated clustering is essential for the subsequent stratified bootstrap process.
The stratified bootstrap method in this research operates by drawing repeated samples from each stratum formed by the DEC algorithm, ensuring a comprehensive data analysis. This technique is effective in overcoming the challenges posed by the spatial distribution of firms, which can vary significantly in an area like Lombardy, known for its diverse economic landscape.
Working with the DEC-derived clusters, the stratified bootstrap is used to compute the distribution of correlation coefficients among various business characteristics like productivity, business size and female employment. These results are robust against the spatial inhomogeneity inherent in the data, underscoring the effectiveness of deep clustering and stratified bootstrap techniques in spatial analysis.
Overall, this paper presents an innovative approach to understanding the high-tech business landscape in a region as economically diverse as Lombardy. Integrating deep clustering with stratified bootstrapping offers valuable insights into the spatial dynamics of business clusters, transcending traditional methods.
Our model considers the characteristics of businesses like their latitude and longitude, therefore it takes into account the geographical distance between the firms but it also takes into account several other characteristics like their economical performances and their sector of activity. This feature is essential to our analysis since it allowed us to create clusters that effectively mitigated the issues brought about by geographical inhomogeneity.
The DEC algorithm's role is crucial as it efficiently segments high-tech firms into coherent groups based on factors like sector activity and economic performance. This segmentation is not merely based on geographical proximity but also deeper, often hidden, interactions of business characteristics. Such a nuanced approach to clustering provides a more accurate representation of the high-tech business environment in Lombardy. This sophisticated clustering is essential for the subsequent stratified bootstrap process.
The stratified bootstrap method in this research operates by drawing repeated samples from each stratum formed by the DEC algorithm, ensuring a comprehensive data analysis. This technique is effective in overcoming the challenges posed by the spatial distribution of firms, which can vary significantly in an area like Lombardy, known for its diverse economic landscape.
Working with the DEC-derived clusters, the stratified bootstrap is used to compute the distribution of correlation coefficients among various business characteristics like productivity, business size and female employment. These results are robust against the spatial inhomogeneity inherent in the data, underscoring the effectiveness of deep clustering and stratified bootstrap techniques in spatial analysis.
Overall, this paper presents an innovative approach to understanding the high-tech business landscape in a region as economically diverse as Lombardy. Integrating deep clustering with stratified bootstrapping offers valuable insights into the spatial dynamics of business clusters, transcending traditional methods.
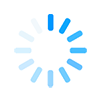