Terceira-S05-S1 Machine Learning in Regional Science: Perspectives, Methods, and Applications
Tracks
Special Session
Wednesday, August 28, 2024 |
16:45 - 18:30 |
S02 |
Details
Chair: Kevin Credit, National Centre for Geocomputation, Maynooth, Ireland; Katarzyna Kopczewska, University of Warsaw, Poland
Speaker
Dr. Mennan Guder
Post-Doc Researcher
Cranfield University
Ethnicity and access to digital energy, health and housing services: A data-driven approach
Author(s) - Presenters are indicated with (p)
Mennan Guder(p), Nazmiye Balta-Ozkan (p)
Discussant for this paper
Ana Luisa Barbosa
Abstract
While urban and regional inequalities on economic growth have been well documented, whether digitisation of services may help to mitigate or exacerbate these gaps is not answered yet. The benefits of digitization vary from more efficient utilisation of utilization of assets to responding to disruptions more efficiently to the design and delivery of more in-demand services. Yet, how these benefits are distributed across the end users (i.e. public) vs service providers vary across the sectors. In the UK, delivery of social housing and primary health care services are universal. While by private companies or local authorities may provide the former, the latter is provided by private companies. Energy services are provided by private actors. This variation in service providers reveal a large degree of variation in types of services and their delivery modes (e.g. websites, apps). One may argue that ensuring equitable access to digital services can be important in addressing urban and regional inequalities where this study is poised to make contributions.
This study develops an agent-based model (ABM) to characterise and analyse minority ethnic communities’ satisfaction with the delivery of online services in health, housing, and energy sectors. The study is part of UK Research and Innovation funded project, Protecting Minority Ethnic Communities Online.
A key innovation of the study is the integration of machine learning (ML) to analyse survey data (N=878) in defining the agents’ attributes and decision parameters. Traditional ABMs rely on rule-based decision parameters, whereas the use of ML allows for the unbiased derivation of rules and enhances simulation outcomes.
By leveraging ML, the model forecasts the online service experience of individual users based on specific attributes such as demographics, health, housing, and energy-related factors, technology usage, and online service preferences and usage patterns. Additionally, the model considers environmental factors such as digital service quality, user-friendliness of digital platforms, staff attitudes, and regional policies. Through the analysis of these attributes and environmental factors, the model predicts online service satisfaction levels within diverse regional contexts.
The developed ABM can then be further used to run different simulations to assess the impact of alternative policy interventions. In conclusion, this research underscores the dual role of ABM and ML in informing policy decisions and guiding service providers to address regional issues effectively. By examining regional variations in online service perception, policymakers and researchers can develop targeted interventions to promote digital inclusion and address socio-economic disparities across diverse communities.
This study develops an agent-based model (ABM) to characterise and analyse minority ethnic communities’ satisfaction with the delivery of online services in health, housing, and energy sectors. The study is part of UK Research and Innovation funded project, Protecting Minority Ethnic Communities Online.
A key innovation of the study is the integration of machine learning (ML) to analyse survey data (N=878) in defining the agents’ attributes and decision parameters. Traditional ABMs rely on rule-based decision parameters, whereas the use of ML allows for the unbiased derivation of rules and enhances simulation outcomes.
By leveraging ML, the model forecasts the online service experience of individual users based on specific attributes such as demographics, health, housing, and energy-related factors, technology usage, and online service preferences and usage patterns. Additionally, the model considers environmental factors such as digital service quality, user-friendliness of digital platforms, staff attitudes, and regional policies. Through the analysis of these attributes and environmental factors, the model predicts online service satisfaction levels within diverse regional contexts.
The developed ABM can then be further used to run different simulations to assess the impact of alternative policy interventions. In conclusion, this research underscores the dual role of ABM and ML in informing policy decisions and guiding service providers to address regional issues effectively. By examining regional variations in online service perception, policymakers and researchers can develop targeted interventions to promote digital inclusion and address socio-economic disparities across diverse communities.
Dr. Kevin Credit
Assistant Professor
Maynooth University
Assessing the causal impact of Chicago’s elevated Bloomingdale Trail on nearby retail activity, construction, and emissions using spatial machine learning
Author(s) - Presenters are indicated with (p)
Kevin Credit (p)
Discussant for this paper
Nazmiye Balta-Ozkan
Abstract
Recently, a growing body of research has emerged to estimate the impact of public transport systems on nearby housing values and new business creation (Credit, 2018; Schmidt et al. 2022; Champagne and Dubé, 2023). However, there is much less work on the local economic impact of new pedestrian and cycling trails, and almost none on the impact of these trails on greenhouse gas emissions reduction, which is an important potential benefit of these investments to environmental sustainability. In addition, the recent development of causal machine learning methods such as the causal forest (Wager and Athey, 2018) provides new opportunities to assess the spatially-heterogenous nature of these causal impacts and generate more useful insights for researchers and policy-makers (Credit and Lehnert, 2023). The goal of this paper is to assess the causal impact of the construction of a new elevated pedestrian and cycling trail in Chicago – called the ‘606’ or Bloomingdale Trail – on nearby retail business activity, building permits, and on-road CO2 emissions. The Bloomingdale Trail presents a useful test case reminiscent of other high-profile rail-trail renovations (such as the BeltLine in Atlanta and the High Line in New York City) that have attracted substantial secondary investment and are touted as ‘sustainable’ engines of local economic development. The paper applies a spatially-specified causal forest on a unique combination of data sources to provide a novel, nuanced, and holistic analysis of the trail’s heterogenous impact on surrounding economic and environmental conditions.
Dr. Debora Gambina
Post-Doc Researcher
University of Palermo
Decoding the Micro-Economic Impact of Carbon Reduction Policy: A Counterfactual Identification via Machine Learning
Author(s) - Presenters are indicated with (p)
Alessandro Cusimano, Federico Fantechi (p), Debora Gambina (p), Fabio Mazzola
Discussant for this paper
Kevin Credit
Abstract
This paper critically examines the effectiveness of contemporary EU environmental and carbon reduction policies, focus of the recent shift towards technology-led initiatives that encourage SMEs to optimize the use or reuse of water, energy, and materials. The complexity of these policies is highlighted, as their success hinges on the active participation of economic actors, transforming a global, public problem into a series of solutions mediated by local, private, economic actors. The paper investigates whether implementing these publicly financed Circular Economy (CE) and green practices and technologies have an economic impact on adopters, a topic that current literature does not conclusively address. The authors aim to contribute to this discussion by investigating the microeconomic impact of varying levels of public support on firms implementing CE and green technologies for carbon reduction. To answer the research question, the authors propose a novel design for counterfactual identification that leverages Machine Learning methods and algorithms. The policy impact over different levels of treatment (public financial support/total tangible assets of the firm) is measured via a Multiple Treatment Matching Difference in Difference model. This model allows for the estimation of the causal impact of a differentiated treatment by comparing the difference in output with untreated units for each level of treatment. The novelty of our contribution lies in the identification of counterfactuals, which is achieved through the use of Machine Learning algorithms. This study, therefore, not only contributes to the understanding of EU environmental policies but also introduces a novel methodological approach to policy impact analysis. The study finds that the impact produced by different levels of treatment forms an inverted 'U' shape. Overall, the produced results have both environmental and microeconomic implication where is shown that the implementation of these technologies can improve the efficiency of production systems with varying intensity.
Ms Ana Luisa Barbosa
Senior Researcher
European Commission - Joint Research Centre
Enhancing European Land Use and Land Change Models with Spatial Machine Learning
Author(s) - Presenters are indicated with (p)
Ana Luisa Barbosa (p), Ana Klinnert, Erik Oudejans, Maarten Hilferink , Ignacio Perez-Dominguez
Discussant for this paper
Federico Fantechi
Abstract
In the rapidly evolving domain of Land Use and Land Change (LULC) studies, adopting advanced predictive tools like Machine Learning (ML) might become increasingly significant. This paper explores the role of Random Forest (RF), in refining the calibration of LULC models by providing additional suitability factors and compares it to Linear Regression (LR) without these RF factors, a commonly used model in this field. Employing a comprehensive, large-scale study across Europe, we leverage RF to calibrate a land-use model incorporating both biophysical variables and socio-economic variables. The results aim to illuminate the benefits and limitations of these two techniques in LULC modeling.
Our research underscores the robust capacity of RF to handle overfitting, manage high-dimensional spaces, and maintain model accuracy whilst providing necessary transparency through stage 1 and stage 2 validation procedures – vital elements in the intricate field of LULC models. The comparison with LR reveals RF's distinct superiority in ranking the importance of identifying the key biophysical and socio-economic factors that affect land use change. Furthermore, RF shows superior performance for non-linear relationships and interaction effects often prevalent in LULC models.
This comparative study intends to bridge the knowledge gap between spatial ML and LULC analysis, inspiring inter-disciplinary dialogues and equipping researchers with precise, efficient tools to evaluate the performance of models with higher complexity. By showcasing RF's superiority as an additional tool to traditionally used LR in LULC modeling, while providing an in-depth understanding of model performance, we highlight the potential of adopting sophisticated ML tools for more accurate prediction without sacrificing transparency.
Discover how integrating advanced ML tools with LULC research can foster more sustainable land-use policies on a global scale.
Our research underscores the robust capacity of RF to handle overfitting, manage high-dimensional spaces, and maintain model accuracy whilst providing necessary transparency through stage 1 and stage 2 validation procedures – vital elements in the intricate field of LULC models. The comparison with LR reveals RF's distinct superiority in ranking the importance of identifying the key biophysical and socio-economic factors that affect land use change. Furthermore, RF shows superior performance for non-linear relationships and interaction effects often prevalent in LULC models.
This comparative study intends to bridge the knowledge gap between spatial ML and LULC analysis, inspiring inter-disciplinary dialogues and equipping researchers with precise, efficient tools to evaluate the performance of models with higher complexity. By showcasing RF's superiority as an additional tool to traditionally used LR in LULC modeling, while providing an in-depth understanding of model performance, we highlight the potential of adopting sophisticated ML tools for more accurate prediction without sacrificing transparency.
Discover how integrating advanced ML tools with LULC research can foster more sustainable land-use policies on a global scale.
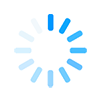