Terceira-G39-O2 Big Data and Regional Science
Tracks
Ordinary/Refereed
Friday, August 30, 2024 |
14:30 - 16:15 |
S07 |
Details
Chair: Raquel Ortega Argiles, University of Manchester, United Kingdom
Speaker
Mr Michał Künstler
Ph.D. Student
University Of Warsaw
Beyond the mean: exploration of night time light intensity based features in regional economic prosperity prediction
Author(s) - Presenters are indicated with (p)
Michał Künstler (p), Piotr Wójcik
Discussant for this paper
Miroslav Despotovic
Abstract
This research delves into the effective use of VIIRS night-time lights intensity (NTLI) data in economic modelling, focusing on designing features to enhance the applicability of NTLI data across academic domains. The study posits that a set of variables can be derived from NTLI data which significantly explains economic prosperity, across spatial, temporal, and regional size dimensions. It further hypothesises that developing separate models for urban and rural regions provides enhanced explanatory power compared to combined models, addressing nuances in the relationship between NTLI and economic prosperity. Lastly, the research will explore how the different types of models, such as decision tree based models, neural networks and others fit the problem of using NTLI data for predicting economic prosperity.
Motivated by the goal of optimizing VIIRS data use for economic modelling, the study explores alternative feature design methods. Economic prosperity is chosen as the focal point due to its prevalence in VIIRS applications and the widespread availability of economic data. The research questions the conventional practice of developing separate models for urban and rural regions and explores the relationship between economic prosperity and various distribution descriptors and variables, such as NTLI distribution moments and scores assigned by convolutional neural networks.
To achieve its primary goal of exploring the stability of importance and relationships across different features over time, sample size, and geographical locations, the study will utilize explainable AI tools. Temporal analysis spans several years between 2012 and 2023. The geographical scope covers various EU countries, such as Poland, Germany and France, due to the consistency in economic data sourcing methods and administrative region definitions. Region size analysis encompasses NUTS 2, NUTS 3, LAU 1, and LAU 2 levels. The mean standardised features’ utility is verified through Shapley Values, with further exploration using Partial Dependence Profiles for the most important features as recognised by a collection of model architectures, including OLS, XGBoost, Random Forest, Neural Networks and SVM. This research aims to contribute to the broader field of spatial and economic modelling by enhancing understanding of the relationship between VIIRS NTLI based features and economic prosperity, as well as by providing researchers with a base set of features more elaborate than the usually utilised arithmetic mean.
Motivated by the goal of optimizing VIIRS data use for economic modelling, the study explores alternative feature design methods. Economic prosperity is chosen as the focal point due to its prevalence in VIIRS applications and the widespread availability of economic data. The research questions the conventional practice of developing separate models for urban and rural regions and explores the relationship between economic prosperity and various distribution descriptors and variables, such as NTLI distribution moments and scores assigned by convolutional neural networks.
To achieve its primary goal of exploring the stability of importance and relationships across different features over time, sample size, and geographical locations, the study will utilize explainable AI tools. Temporal analysis spans several years between 2012 and 2023. The geographical scope covers various EU countries, such as Poland, Germany and France, due to the consistency in economic data sourcing methods and administrative region definitions. Region size analysis encompasses NUTS 2, NUTS 3, LAU 1, and LAU 2 levels. The mean standardised features’ utility is verified through Shapley Values, with further exploration using Partial Dependence Profiles for the most important features as recognised by a collection of model architectures, including OLS, XGBoost, Random Forest, Neural Networks and SVM. This research aims to contribute to the broader field of spatial and economic modelling by enhancing understanding of the relationship between VIIRS NTLI based features and economic prosperity, as well as by providing researchers with a base set of features more elaborate than the usually utilised arithmetic mean.
Mr Emanuele Calò
Ph.D. Student
IMT Lucca
Multidimensional Territorial Attractiveness: an Application to European Flows
Author(s) - Presenters are indicated with (p)
Emanuele Calò (p), Angelo Facchini
Discussant for this paper
Michał Künstler
Abstract
The attractiveness of regions and territories for firms, investments, tourists, students, talented people, and other categories is a relevant issue for regional economic development. Usually, studies on this topic have focused only on one of the types of flows concerned. That is, these dimensions of territorial attractiveness have been studied separately and partially [1]. For example, many studies have been conducted only on the territorial attractiveness for foreign direct investments (FDIs) or tourists. However, this topic has rarely been approached and studied using a comprehensive analytical strategy.
The research question we studied is thus the following: from a multidimensional point of view (i.e., dealing with different types of flows), what makes a region attractive, and to whom?
The data we have used comes from the ESPON project [2]. Specifically, region-to-region (NUTS 2) origin-destination (OD) matrices for People Tourism, People Migration, Freight of Goods by transport mode, Capital FDI, Knowledge Erasmus students, People Passengers by transport mode, Capital Remittances, Knowledge H2020.
We decided to use Network Science tools to characterize these flows properly. Indeed, we have built a multilayer network where each layer is a weighted directed network representing a different flow type and studied its basic statistics (assortativity, clustering, centrality measures, etc.). Then, we compared the results with null models to find significant properties emerging from the data that the models cannot explain.
References:
[1] Musolino, D., & Kotosz, B. (2023). A new territorial attractiveness index at the international scale: design, application and patterns in Italy. The Annals of Regional Science, 1-29.
[2] Russo, A., Smith, I., Atkinson, R., Servillo, L. A., Madsen, B., & Van den Borg, J. (2012). ATTREG. The Attractiveness of European regions and cities for residents and visitors-Scientific Report.
The research question we studied is thus the following: from a multidimensional point of view (i.e., dealing with different types of flows), what makes a region attractive, and to whom?
The data we have used comes from the ESPON project [2]. Specifically, region-to-region (NUTS 2) origin-destination (OD) matrices for People Tourism, People Migration, Freight of Goods by transport mode, Capital FDI, Knowledge Erasmus students, People Passengers by transport mode, Capital Remittances, Knowledge H2020.
We decided to use Network Science tools to characterize these flows properly. Indeed, we have built a multilayer network where each layer is a weighted directed network representing a different flow type and studied its basic statistics (assortativity, clustering, centrality measures, etc.). Then, we compared the results with null models to find significant properties emerging from the data that the models cannot explain.
References:
[1] Musolino, D., & Kotosz, B. (2023). A new territorial attractiveness index at the international scale: design, application and patterns in Italy. The Annals of Regional Science, 1-29.
[2] Russo, A., Smith, I., Atkinson, R., Servillo, L. A., Madsen, B., & Van den Borg, J. (2012). ATTREG. The Attractiveness of European regions and cities for residents and visitors-Scientific Report.
Dr. Miroslav Despotovic
Full Professor
University Of Applied Sciences Kufstein Tirol
Multitasking Modeling for Attractiveness Assessment of Austrian Landscapes Using Complementary and Social Media Data
Author(s) - Presenters are indicated with (p)
Miroslav Despotovic (p), Christoph Hauser
Discussant for this paper
Emanuele Calò
Abstract
The assessment of ecosystems is becoming increasingly important in science, driven by the discourse on their intrinsic value, aesthetics and characteristics contained in landscape value typologies, from which recreational values and intangible benefits of such systems can be derived. The underlying problem is to methodically quantify such aesthetic values and determine the associated proxies, as the aesthetic values of landscapes and the recreational value in the natural environment can be influenced by various landscape characteristics, but also by socio-economic or cultural characteristics. A promising and increasingly popular alternative to quantifying landscape aesthetics is the application of geocoded data from social media. We investigate the potential of geographically located social media content for the spatial quantification of aesthetic values of Austrian landscapes posted on social media platforms by leisure- and sports-active users. To this end, we regress different types of aesthetic values in a multi-task deep learning model using landscape, socio-economic and contextual variables. In contrast to previous studies, we use aggregated small-scale data ( base grid of 100 m²) for the socio-economic variables and a multi-output setup with cross-validation of the data for the modeling. This enables the aesthetics values to be inferred for the entire study area. Furthermore, we use the small-scale data to investigate the extent to which a separation of local versus foreign visitors can be inferred (e.g. through sports activity or whether there is an effect through local sports enthusiasts). The research shows promising results as well as indications of local influencing factors and areas with potential. We believe that this study provides new insights into the use of alternative data and analytical techniques to quantify landscape values and provides a promising basis for further research in this area.
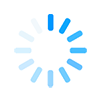