Online-G42 Quantitative spatial analysis
Tracks
Ordinary Session
Tuesday, August 27, 2024 |
14:30 - 16:15 |
Details
Chair: Daniel Felsenstein
Speaker
Ms Yilin Chen
Ph.D. Student
Nagoya University
Can higher-quality nighttime lights predict sectoral GDP across subnational regions? Urban and rural luminosity across the provinces in Türkiye
Author(s) - Presenters are indicated with (p)
Yilin Chen (p), Uğur Ursavaş, Carlos Mendez
Discussant for this paper
Manfred M. Fischer
Abstract
We investigate the potential of higher-quality nighttime light (NTL) data for predicting the sectoral gross domestic product (GDP) across subnational regions. Specifically, we use satellite images from the Visible Infrared Imaging Radiometer Suite (VIIRS) to study the relationship between regional luminosity and sectoral production across 81 provinces in Türkiye over the 2004-2020 period. Luminosity intensity is further decomposed into urban and rural components using land cover data. Employing pooled ordinary least squares, between-estimator, and within-estimator regressions, we examine the relationship between NTL and total GDP, agricultural GDP, non-agricultural GDP, industrial GDP, and services GDP. Our results show that urban NTL exhibits the most robust correlation with non-agricultural GDP. Notably, industrial GDP shows the highest GDP-NTL elasticity, pointing out the high predictive performance of urban NTL. From an estimation standpoint, the between-estimator yields superior predictive performance and more robust GDP-NTL correlations compared to other models. We also find that after accounting for spatial and temporal fixed effects, the within-estimator does not identify a significant relationship between NTL and GDP. Thus, we conclude by arguing that higher-quality nighttime light (NTL) data accurately predict sectoral GDP differences across regions but have weaker predictive power for annual GDP changes.
Prof. Mario Intini
Assistant Professor
University of Bari
Application of machine learning algorithms to quality-of-life decision-making problems
Author(s) - Presenters are indicated with (p)
Angela Stefania Bergantino, Mario Intini (p), Michele Vitagliano
Discussant for this paper
Yilin Chen
Abstract
This paper identifies the main drivers contributing to increasing territorial gaps and does not guarantee homogeneous quality standards between Italian provinces (NUTS-3) using machine learning (ML) models. The provincial quality of life indicators (LQI) developed by Il Sole 24 Ore is the variable of interest in this paper. LQI aims to provide researchers and policymakers with a guideline to understand how perceived quality differs within a country and across provinces over time. This paper, using a homogeneous mix of data certified by institutional sources and web scraping techniques, aims to improve the classification or prediction problems but also wants to use several ML techniques to provide an interpretable tool for decision-makers through decision-making tree representation. Results will explain the main factors that improve the perceived quality of life in countering youth migration from inner areas, addressing gender disparities, and understanding the relationships with climate change. For these reasons, results will provide planners and decision-makers with easily interpretable results and useful econometric tools.
Prof. Carlos Mendez
Associate Professor
Nagoya University
Nighttime Lights and Local Economic Activity: Evidence from Developed and Developing Countries
Author(s) - Presenters are indicated with (p)
Carlos Mendez (p), Yilin Chen, Hussein Suleiman
Discussant for this paper
Mario Intini
Abstract
Satellite nighttime lights (NTL) are commonly used for evaluating and validating the economic performance of national and subnational economies. However, many studies in the economics literature rely on outdated and imprecise Defense Meteorological Satellite Program (DMSP) data. This study employs newly released and higher-resolution nightlight images from the Visible Infrared Imaging Radiometer Suite (VIIRS). Our objective is to evaluate and validate the performance of 1726 subnational economies across developed and developing countries. Consistent with the emerging evidence from single-country analyses, our results from multi-country analyses are threefold. First, when compared to (simulated) DMSP data, VIIRS data provide a more accurate prediction of economic activity across both developed and developing countries. Second, nighttime light data are more useful for predicting differences across economies than changes over time within economies. Third, DMSP data understates spatial inequality across both developed and developing countries. Overall, these results point out that as geospatial measurement technologies advance, so too will our understanding of economic activity.
Prof. Daniel Felsenstein
Full Professor
Hebrew University of Jerusalem
The joint determination of morbidity and vaccination in the spatiotemporal analysis of Covid-19
Author(s) - Presenters are indicated with (p)
Daniel Felsenstein (p), Michael Beenstock, Matan Gdaliahu
Discussant for this paper
Carlos Mendez
Abstract
This paper examines the mutual dependence between Covid-19 morbidity and vaccination rollout. A theory of endogenous immunization is proposed in which the decision to become vaccinated varies directly with the risks of contagion, and the public self-selects into self-protection. Hence, Covid-19 morbidity varies inversely with vaccination rollout, and vaccination rollout varies directly with Covid-19 morbidity. The paper leverages the natural sequencing between morbidity and immunization to identify the causal order in the dynamics of this relationship. A modified SIR model is estimated using spatial econometric methods for weekly panel data for Israel at a high level of spatial granularity. Connectivity between spatial units is measured using physical proximity and a unique mobility-based measure. Spatiotemporal models for morbidity and vaccination rollout show that not only does morbidity vary inversely with vaccination rollout, vaccination rollout varies directly with morbidity. The utility of the model for public health policy targeting, is highlighted.
Prof. Manfred M. Fischer
Full Professor
Vienna University of Economics and Business
Spatial mismatch revisited using mobile phone data and spatial econometric tools
Author(s) - Presenters are indicated with (p)
Manfred.M. Fischer (p), Umut Türk, Marina Toger, John Östh
Discussant for this paper
Daniel Felsenstein
Abstract
The COVID-19 pandemic exacerbated job accessibility and spatial mismatch challenges. Higher-income workers often shifted to remote work, while less qualified, lower-income workers faced commuting challenges or job loss. This study primarily aims to investigate the determinants of commuting mobility, utilising mobile phone data to trace commuter movements in the greater Stockholm area across five years (2019-2023 on Thursdays in March). We create OD matrices to map connections between residential neighbourhoods and workplaces before, during, and after the pandemic.
While our main goal is to understand the factors influencing commuting mobility throughout this period, our research also explores two additional aspects: i) the extent of spatial mismatch and its evolution during the pandemic, and ii) the impact of the rise in distance working on commuting behaviour, particularly whether it reverted to pre-pandemic patterns in the post-pandemic period and how spatial mismatch was manifested during this transition. The study employs spatial Durbin panel modelling with Bayesian estimation to address the primary objective of identifying the determinants of commuting mobility. To provide an initial descriptive context, particularly for examining spatial mismatch and the impact of distance working on commuting behaviours, we undertake a concise exploratory study and employ Geographic Information Systems (GIS).
While our main goal is to understand the factors influencing commuting mobility throughout this period, our research also explores two additional aspects: i) the extent of spatial mismatch and its evolution during the pandemic, and ii) the impact of the rise in distance working on commuting behaviour, particularly whether it reverted to pre-pandemic patterns in the post-pandemic period and how spatial mismatch was manifested during this transition. The study employs spatial Durbin panel modelling with Bayesian estimation to address the primary objective of identifying the determinants of commuting mobility. To provide an initial descriptive context, particularly for examining spatial mismatch and the impact of distance working on commuting behaviours, we undertake a concise exploratory study and employ Geographic Information Systems (GIS).
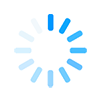