G08-O7 Regional Competitiveness, Innovation and Productivity
Tracks
Refereed/Ordinary Session
Friday, August 30, 2019 |
9:00 AM - 10:30 AM |
UdL_Room 105 |
Details
Chair: Reinhold Kosfeld
Speaker
Dr. David Comerford
University Lecturer
University Of Strathclyde
The Wealth of Cities
Author(s) - Presenters are indicated with (p)
David Comerford (p)
Abstract
Modern trade models (Arkolakis et al, 2012) provide micro-foundations for the empirical observation that city size and productivity are positively related. Agglomeration benefits in these models arise from a love of variety in consumption, or from a value of specialisation in production, with larger markets allowing a larger suite of consumption goods or intermediate inputs. The effective returns to scale, and hence size of agglomeration effects, in these models can be calibrated using spatial price differences (Simonovska & Waugh, 2014) or macroeconomic correlations across the business cycle (Backus et al, 1994). Typically however, these models have a constant value for the parameter (substitution elasticity) thus estimated, so that a doubling of city size always has the same proportionate benefit to average productivity whether it is a large city or a small city that we are doubling.
Perhaps a more reasonable hypothesis is that this substitution elasticity is itself a function of scale. Following Zhelobodko et al (2012), we consider a variable elasticity of substitution version of the Krugman (1980) model, and calibrate it to the more than 300 Metropolitan Statistical Areas (MSAs) in the US. Using US data controls for institutional differences across units of observation. We control for observed differences in human capital levels, and assume that trade flows between cities are given by a gravity relationship (assuming trade costs are proportional to physical distance). MSAs can have a large economic size either by being large, or by being well connected. What ‘substitution elasticity - economic size’ relationship allows the model to best fit the observed pattern of economic size and per capita output without relying on an unobserved TFP residual?
We find evidence that supports what Zhelobodko et al (2012) refer to as “increasing relative love for variety (RLV)". Increasing RLV generates pro-competitive effects as market size increases which means that agglomeration benefits are exhausted as cities become very large. This has important policy implications as it suggests that focusing intervention on creating scale for small populations is potentially more valuable than further investments to increase market size in the largest population centres.
Perhaps a more reasonable hypothesis is that this substitution elasticity is itself a function of scale. Following Zhelobodko et al (2012), we consider a variable elasticity of substitution version of the Krugman (1980) model, and calibrate it to the more than 300 Metropolitan Statistical Areas (MSAs) in the US. Using US data controls for institutional differences across units of observation. We control for observed differences in human capital levels, and assume that trade flows between cities are given by a gravity relationship (assuming trade costs are proportional to physical distance). MSAs can have a large economic size either by being large, or by being well connected. What ‘substitution elasticity - economic size’ relationship allows the model to best fit the observed pattern of economic size and per capita output without relying on an unobserved TFP residual?
We find evidence that supports what Zhelobodko et al (2012) refer to as “increasing relative love for variety (RLV)". Increasing RLV generates pro-competitive effects as market size increases which means that agglomeration benefits are exhausted as cities become very large. This has important policy implications as it suggests that focusing intervention on creating scale for small populations is potentially more valuable than further investments to increase market size in the largest population centres.
Ms Ana Gomez-Loscos
Senior Researcher
Bank of Spain
What drives regional business cycles in Europe?
Author(s) - Presenters are indicated with (p)
Ana Gomez-Loscos (p), Eduardo Bandres , Lola Gadea
Abstract
The aim of this paper is twofold. First, to identify regional business cycles in Europe, obtaining datings of business cycles that allow us to identify possible groups of regions and to assess how interdependence in European regions has changed over time, paying special attention both to the euro cash changeover and the global financial crisis. Second, to determine the driving forces behind each group of regions, that is, to identify the economic, cultural and social factors that may help explain regional business cycles. We identify five different groups of European regions. Moreover, we find an increase over time in regional business cycle synchronization. We detect that the variables that play the most important role to identify groups of regions are those related to well-being and geography and culture.
Prof. Reinhold Kosfeld
Associate Professor
University of Kassel
The role of R&D-intensive clusters in regional competition and employment development
Author(s) - Presenters are indicated with (p)
Reinhold Kosfeld (p), Timo Mitze
Abstract
Modern cluster theory provides reasons for positive external effects that may accrue from interaction in a group of proximate enterprises operating in common and related fields. Especially Porter externalities attribute competitive advantages to innovative clusters that are characerized by favourable demand and factor conditions, rivalry and the presence of related and supporting industries. While Porters diamond model substantiates a close link of regional competition to productivity growth, the effect on employment development are less clear-cut.
There is a lot of empirical research to reveal cluster effects on economic performance. Recently, the papers of Delgado, Porter and Stern (2014) and Slaper, Harmon and Rubin (2018) address this issue from different points of view. Delgado, et al. (2014) evaluate the role of clusters on regional industry performance in the interplay between dispersion and agglomerating forces, whereas Slaper et al. (2018) differentiate between effects of cluster strength and diversity on regional performance without regard to potential countervailing forces. While the former paper focuses on growth of employment and innovation, the latter investigates cluster impacts on productivity, production, compensation and income growth. Both approaches identify regional clusters with the aid of traditional instruments like input-output analysis and the location quotient. The studies exploit data from the US Cluster Mapping Project.
The present paper focusses on impacts of R&D-intensive industry clusters on regional competition and employment development. To account for both dispersion and agglomeration forces we draw on the convergence model of regional growth (Islam 1995; Sala-I-Martin 1996). Within this model we differentiate between effects of cluster strength and diversity as counterparts of related and unrelated variety hypothesized by Frenken et al. (2007) at the industry level. The identification of R&D clusters is based on an hybrid approach of qualitative input-output analysis and spatial scanning (Kosfeld and Titze 2017).
The cluster impact analysis is conducted for a panel of German NUTS-3 regions in the period 2002 -2012. In line with Porter’s diamond model (Porter 2000), competition is operationalzied by productivity growth. Controling for regional characteristics, a significant cluster strength effect is found in conjuncction with conditional convergence. When both cluster variables strength and diversity enter the panel model the isolated diversification effect vanishes. In a more detailed analysis it is shown that productivity growth is strongly related to pharmaceutical clusters and electrical machinery clusters. This corroborates the attribution of growth effects to special types of clusters found by Maine, Shapiro and Vining (2008).
There is a lot of empirical research to reveal cluster effects on economic performance. Recently, the papers of Delgado, Porter and Stern (2014) and Slaper, Harmon and Rubin (2018) address this issue from different points of view. Delgado, et al. (2014) evaluate the role of clusters on regional industry performance in the interplay between dispersion and agglomerating forces, whereas Slaper et al. (2018) differentiate between effects of cluster strength and diversity on regional performance without regard to potential countervailing forces. While the former paper focuses on growth of employment and innovation, the latter investigates cluster impacts on productivity, production, compensation and income growth. Both approaches identify regional clusters with the aid of traditional instruments like input-output analysis and the location quotient. The studies exploit data from the US Cluster Mapping Project.
The present paper focusses on impacts of R&D-intensive industry clusters on regional competition and employment development. To account for both dispersion and agglomeration forces we draw on the convergence model of regional growth (Islam 1995; Sala-I-Martin 1996). Within this model we differentiate between effects of cluster strength and diversity as counterparts of related and unrelated variety hypothesized by Frenken et al. (2007) at the industry level. The identification of R&D clusters is based on an hybrid approach of qualitative input-output analysis and spatial scanning (Kosfeld and Titze 2017).
The cluster impact analysis is conducted for a panel of German NUTS-3 regions in the period 2002 -2012. In line with Porter’s diamond model (Porter 2000), competition is operationalzied by productivity growth. Controling for regional characteristics, a significant cluster strength effect is found in conjuncction with conditional convergence. When both cluster variables strength and diversity enter the panel model the isolated diversification effect vanishes. In a more detailed analysis it is shown that productivity growth is strongly related to pharmaceutical clusters and electrical machinery clusters. This corroborates the attribution of growth effects to special types of clusters found by Maine, Shapiro and Vining (2008).
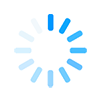