Alicante-G16 Smart Specialization
Tracks
Refereed/Ordinary Session
Wednesday, August 30, 2023 |
14:30 - 16:15 |
0-C01 |
Details
Chair: Monica Mihaela Tudor
Speaker
Dr. Ilyes Boumahdi
Post-Doc Researcher
National Institute of Statistics and Applied Economics
Smart specialization: New industrialization paths for Casablanca
Author(s) - Presenters are indicated with (p)
Ilyes Boumahdi (p), Nouzha Zaoujal
Discussant for this paper
Monica Mihaela Tudor
Abstract
The research for the renewal of the productive system of the industrial lung of Morocco, namely the region of Casablanca-Settat (CS), requires alternative quantitative bases. Also, we propose to adapt the smart specialization framework to CS based on their relatedness density (Hidalgo et al., 2018) and their level of complexity (Hidalgo & Hausmann, 2009) as developed in the context of support for innovation (P.-A. Balland et al., 2019). For this, we use data relating to the exports, in 2015, of nearly 8000 establishments by region of Morocco (12) and industrial activities (223) by using the Econgeo R package (P. A. Balland, 2017).
Thus, CS has exhausted all the possibilities of the “high-road” policy and can only qualify for it through a greenfield establishment of companies in the same way as the former industrial regions (Example of Lancashire). On the other hand, two activities (transformation of tea and coffee and manufacture of plaster) can be supported in a logic of strategic betting (“Casino” policy with high expected gain but at a cost which is also high).
Moreover, CS can develop in a logic of parsimonious strategy along the activities of the “slow-road” policy by supporting existing companies, and which already export in these activities but without having a relative comparative advantage, or those to be brought out (Aluminum metallurgy; treatment and coating of metals; manufacture of electrical equipment, etc.). On the other hand, CS would engage in a “dead-end” policy by supporting the activities of the south-west quadrant (processing and preservation of vegetables, production of refreshing drinks, manufacture of cement, etc.) linked to a low expected gain coupled with a high cost.
Thus, CS presents the widest portfolio of potential industrial smart specialization activities (21 activities outside the "dead-end" policy) and very diversified in the same way as the regional hubs (Example of Ile-de-France). This reveals the capacity of the economic capital region to broaden its spectrum of industrial specialization either in a parsimonious way with prospects for growth and gradual valuation but coupled with low risk and cost or in the form of a strategic bet with growth prospects and high valuation but coupled with high risk and cost (Example of Noord-Brabant region).
Thus, CS has exhausted all the possibilities of the “high-road” policy and can only qualify for it through a greenfield establishment of companies in the same way as the former industrial regions (Example of Lancashire). On the other hand, two activities (transformation of tea and coffee and manufacture of plaster) can be supported in a logic of strategic betting (“Casino” policy with high expected gain but at a cost which is also high).
Moreover, CS can develop in a logic of parsimonious strategy along the activities of the “slow-road” policy by supporting existing companies, and which already export in these activities but without having a relative comparative advantage, or those to be brought out (Aluminum metallurgy; treatment and coating of metals; manufacture of electrical equipment, etc.). On the other hand, CS would engage in a “dead-end” policy by supporting the activities of the south-west quadrant (processing and preservation of vegetables, production of refreshing drinks, manufacture of cement, etc.) linked to a low expected gain coupled with a high cost.
Thus, CS presents the widest portfolio of potential industrial smart specialization activities (21 activities outside the "dead-end" policy) and very diversified in the same way as the regional hubs (Example of Ile-de-France). This reveals the capacity of the economic capital region to broaden its spectrum of industrial specialization either in a parsimonious way with prospects for growth and gradual valuation but coupled with low risk and cost or in the form of a strategic bet with growth prospects and high valuation but coupled with high risk and cost (Example of Noord-Brabant region).
Dr. Alexander Kaiser
Post-Doc Researcher
Universität der Bundeswehr München
Evaluating the relationship of urban characteristics with the productivity of Higher Education Institutions.
Author(s) - Presenters are indicated with (p)
Alexander Kaiser (p), Axel Schaffer
Discussant for this paper
Ilyes Boumahdi
Abstract
While the importance of higher education institutions (HEI) for the economic development and innovative power of cities and adjacent regions has long been established in the literature, little is known about how different urban environments relate to institutionally differing characteristics of HEI and i.e., their positioning in university rankings. The latter might be due to commonly analyzed data sets (e.g., ETER or Aquameth database) providing data on the aggregate national level or general administrative data (such as total staff or amount of funding) and thus do not allow for a consideration of individual intra-institutional settings and their embedding into an urban environment. Given the growing importance of tackling complex scientific research issues in inter- or transdisciplinary groups of researchers, one factor that has just recently received increasing attention by economists of science is the so-called division of cognitive labor. The latter supposes an efficient allocation of researcher specialization due to scientists' credit maximizing behavior. To the best of our knowledge, we are the first to have compiled a data set that allows for an empirical examination of this hypothesis, collecting specialization data of 20 universities regularly listed in renowned university rankings. The dataset covers the period 1890 to 2020 using HEI's institutional (instead of affiliated publication data) and contains information on individual professorial chairs' research areas and their disciplinarity. In order to measure the effect of specialization on research productivity we employ a nonparametric approach to relate citation output to professorial staff input. We differ from the existing literature by exclusively considering faculties of natural sciences and further differentiate the staff input according to disciplines within the natural sciences. Arguably, such a differentiation results in a granular model set-up that provides a suitable reflection of the substantially differing publication production processes of different scientific disciplines. In a second step, intra-institutional specialization is considered along covariates of local characteristics such as economic performance, access to human capital, country-belonging and native language. Preliminary findings (based on the subsample for 1890 to 1920) suggest that the degree of specialization as well as urban characteristics are important determinants of HEIs productivity.
Dr. Monica Mihaela Tudor
Senior Researcher
Romanian Academy - Institute for Agricultural Economics
Regional smartness and territorial innovation – Romanian case study -
Author(s) - Presenters are indicated with (p)
Monica Mihaela Tudor (p), Elisabeta Rosu, Catalin Munteanu
Discussant for this paper
Alexander Kaiser
Abstract
The paper aims to evaluate, using an innovative methodology, the smartness level of the development regions in Romania, more precisely: the ability of NUTS II regions to capitalize on their human, structural and relational capital and their ability to integrate different actors and territories into regional innovative practices.
The analytical approach was subordinated to the hypothesis that a high level of regional smartness ensures a favourable climate for the development of smart initiatives at the regional level, including in rural areas that operate in close connection with the regional system assembly.
In the regional smartness assessment, a methodology based on Stochastic Multicriteria Acceptability Analysis, advanced within the Horizon 2020 PERCEIVE project, was chosen. By applying the SMAA method, it is possible to determine how intelligent each of the NUTS II regions in Romania is. The regional smartness parameters were analysed in their temporal evolution (in the years 2013 and 2020) in order to evaluate to what extent territorial disparities deepen or are overcome under the influence of different policies and programs that address territorial development.
The results of the study show a positive evolution along the previous EU multiannual framework programme (2013-2020) by reducing the gaps between regions in terms of regional smartness. Therefore, there are favourable premises for intelligent, technology-based growth in all the territories of Romania. However, in the period 2013-2020, some regions recovered the pre-existing gaps regarding the level of intelligence faster (South West, West, South), and others lost the comparative advantages they had at the beginning of the analysed period (North West, North East, Bucharest).
The analytical approach was subordinated to the hypothesis that a high level of regional smartness ensures a favourable climate for the development of smart initiatives at the regional level, including in rural areas that operate in close connection with the regional system assembly.
In the regional smartness assessment, a methodology based on Stochastic Multicriteria Acceptability Analysis, advanced within the Horizon 2020 PERCEIVE project, was chosen. By applying the SMAA method, it is possible to determine how intelligent each of the NUTS II regions in Romania is. The regional smartness parameters were analysed in their temporal evolution (in the years 2013 and 2020) in order to evaluate to what extent territorial disparities deepen or are overcome under the influence of different policies and programs that address territorial development.
The results of the study show a positive evolution along the previous EU multiannual framework programme (2013-2020) by reducing the gaps between regions in terms of regional smartness. Therefore, there are favourable premises for intelligent, technology-based growth in all the territories of Romania. However, in the period 2013-2020, some regions recovered the pre-existing gaps regarding the level of intelligence faster (South West, West, South), and others lost the comparative advantages they had at the beginning of the analysed period (North West, North East, Bucharest).
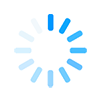