Alicante-G40 Spatial Econometrics
Tracks
Refereed/Ordinary Session
Thursday, August 31, 2023 |
14:30 - 16:15 |
1-B12 |
Details
Chair: Alberto Tidu
Speaker
Dr. Maryna Makeienko
Assistant Professor
Universidad Villanueva/esic
Spatial Trends and Spatial Econometric Structures: Practical application to different context data.
Author(s) - Presenters are indicated with (p)
Maryna Makeienko (p), Mariano Matilla García
Discussant for this paper
Alberto Tidu
Abstract
Spatial trend concept was proved to be useful in order to depict the
systematic variations of the phenomenon concerned over a region based
on geographical locations. We perform the analysis based on use of
geographically restricted semiparametric spatial models on the datasets
with geographical information. This is implemented to check if there exist
potential leading deterministic spatial components and whether we can
econometrically model spatial economic relations that might contain
unobserved spatial structure of unknown form. Hypothesis testing is
conducted with a symbolic-entropy based non-parametric statistical
procedure, proposed in Garcia-Cordoba et al. (2019), which does not rely
on prior weight matrices assumptions. The main question to be responded
is whether this type of models that merely incorporate space coordinates
might be sufficient to capture space dependence when applied to
different types of data. Moreover, we study what intrinsic characteristics
of the economic problem or the dependent variable itself make feasible
(and optimal) to use the methodological approach mentioned.
The analysis is based on six different datasets. Each of the dataset includes
the full information on the object of the analysis, where a certain relation
can be found. Apart from the special characteristics of the units, every
dataset includes the information of the geographical position (longitude
and latitude) of the units described. Thus, we have a possibility to
compare the general characteristics of data analyzed to produce a better
methodology of specifying a trend methodology (including a delta test
usage).
systematic variations of the phenomenon concerned over a region based
on geographical locations. We perform the analysis based on use of
geographically restricted semiparametric spatial models on the datasets
with geographical information. This is implemented to check if there exist
potential leading deterministic spatial components and whether we can
econometrically model spatial economic relations that might contain
unobserved spatial structure of unknown form. Hypothesis testing is
conducted with a symbolic-entropy based non-parametric statistical
procedure, proposed in Garcia-Cordoba et al. (2019), which does not rely
on prior weight matrices assumptions. The main question to be responded
is whether this type of models that merely incorporate space coordinates
might be sufficient to capture space dependence when applied to
different types of data. Moreover, we study what intrinsic characteristics
of the economic problem or the dependent variable itself make feasible
(and optimal) to use the methodological approach mentioned.
The analysis is based on six different datasets. Each of the dataset includes
the full information on the object of the analysis, where a certain relation
can be found. Apart from the special characteristics of the units, every
dataset includes the information of the geographical position (longitude
and latitude) of the units described. Thus, we have a possibility to
compare the general characteristics of data analyzed to produce a better
methodology of specifying a trend methodology (including a delta test
usage).
Mr Julio Cesar Lopes
Senior Researcher
Banco do Brasil SA
Piggybacking on soy: spatial spillovers of soy on GDP per capita income in Brazilian municipalities
Author(s) - Presenters are indicated with (p)
Julio Cesar Lopes (p)
Discussant for this paper
Maryna Makeienko
Abstract
Brazil is the world's largest producer of soy, accounting for approximately 36% of world production, most of which is destined for the foreign market, mainly China. Internally, the grain has consolidated itself as the main product of national agriculture and today corresponds to approximately 50% of all Brazilian agricultural production. Likewise, it is the main product exported by the country and guaranteed the entry of approximately US$ 50 billion last year. These numbers reflect of the rapid expansion of soybean planting in the country over the last few decades. In 1990, there was a record of soy planting in only 21% of Brazilian municipalities, a percentage that jumped to 25% in 2000, 31% in 2010 and 41% in 2020.
This work investigates the effects of soybean production on the local GDP per capita and what are its spatial spillovers over neighboring municipalities between the years 2002 and 2020 using a spatial panel. Preliminary results indicate that, on average, for a 1% increase in the amount of soy produced in a municipality, there is an increase of 0.14% in the local GDP per capita. In terms of spatial spillover, this impact on neighbors is also positive and assumes a value of 0.02%.
The work also investigates the spatial effects of other variables and the results indicate that the expansion of soybean area in a municipality negatively affects the GDP per capita of the neighboring municipality. On the other hand, the spatial spillovers resulting from a greater number of workers with a high school education, as well as those arising from constitutional transfers made by the federal government, are also positive and have a greater impact than those arising from soybean production.
It is important to point out that, at this moment, the work does not investigate the effects of soy expansion on the destruction of natural biomes, such as the Cerrado or the Amazon, a topic for the next steps of the research.
This work investigates the effects of soybean production on the local GDP per capita and what are its spatial spillovers over neighboring municipalities between the years 2002 and 2020 using a spatial panel. Preliminary results indicate that, on average, for a 1% increase in the amount of soy produced in a municipality, there is an increase of 0.14% in the local GDP per capita. In terms of spatial spillover, this impact on neighbors is also positive and assumes a value of 0.02%.
The work also investigates the spatial effects of other variables and the results indicate that the expansion of soybean area in a municipality negatively affects the GDP per capita of the neighboring municipality. On the other hand, the spatial spillovers resulting from a greater number of workers with a high school education, as well as those arising from constitutional transfers made by the federal government, are also positive and have a greater impact than those arising from soybean production.
It is important to point out that, at this moment, the work does not investigate the effects of soy expansion on the destruction of natural biomes, such as the Cerrado or the Amazon, a topic for the next steps of the research.
Dr. Kateryna Zabarina
Assistant Professor
Uniwersytet Warszawski / University of Warsaw, Faculty of Economic Sciences
Application of hybrid Gibbs processes to firm location forecasting
Author(s) - Presenters are indicated with (p)
Kateryna Zabarina (p)
Discussant for this paper
Julio Cesar Lopes
Abstract
Importance of geo-location is obvious for businesses, since it is a key for success (Bhat, Paleti, and Singh 2014, p.462). Forecast of location for a new business plant is difficult, since a lot of things have to be taken into account. As a base of such forecast, one should estimate a model explaining firm locations. Already existing models use aggregated data, which do not seem to be appropriate for such problem, since they do not reflect spatial nature and distribution of data, do not account for spatial factors (such as localization patterns (Pablo-Martí, Muñoz-Yebra, and Santos 2014), issue of economic clusters and agglomeration economies (Buenstorf and Guenther 2007; Lasch, Robert, and Le Roy 2013; Walz and Wellisch 1996; Delgado, Porter, and Stern 2010; Jo and Lee 2014; Basile, Castellani, and Zanfei 2008; J.-M. Arauzo-Carod and Manjón-Antolín 2012; Rosenthal and Strange 2003; Jofre‐Monseny, Marín‐López, and Viladecans‐Marsal 2014)) or existence of spatial dependence and heterogeneity.
Aim of this paper is a development of method, which allows to predict location of a new point and takes into account individual characteristics of point, its neighbours and spatial covariates. Paper can be considered as a contribution to development of spatial micro-econometrics.
Preliminary results show that consideration of spatial factors (such as distance to center or line pattern of roads) and interactions (captured with hybrid Gibbs process) allows to produce a model which passes goodness-of-fit tests and can be used in prediction of new locations.
Aim of this paper is a development of method, which allows to predict location of a new point and takes into account individual characteristics of point, its neighbours and spatial covariates. Paper can be considered as a contribution to development of spatial micro-econometrics.
Preliminary results show that consideration of spatial factors (such as distance to center or line pattern of roads) and interactions (captured with hybrid Gibbs process) allows to produce a model which passes goodness-of-fit tests and can be used in prediction of new locations.
Dr. Izabella Szakálné Kanó
Associate Professor
University of Szeged
Measuring spatial and social inequalities in Hungary between 2010 and 2020 and estimating them using spatial econometrical methods
Author(s) - Presenters are indicated with (p)
Izabella Szakálné Kanó (p), György Vida
Discussant for this paper
Kateryna Zabarina
Abstract
In today's digitalizing global economy, competition between regions and municipalities intensifies across borders. In addition, in the recent period, two world economic crises emerged different ways. One was the global financial crisis, and another was the global social and economic challenges of the Covid-19 pandemic and the Russo-Ukrainian war. The world was characterized by relative economic prosperity in the period between the two shocks. Still, it can be stated that the analyzed regions' competitiveness over time plays an important role in post-crisis recovery. It is not different in the United States, the European Union, and, within it, Hungary. It can be stated that the regional revealed competitiveness in Hungary between 2010 and 2020 showed a characteristic geographical pattern, which needs to be analyzed.
Consequently, the study aims to examine the geographical patterns of competitiveness and income disparities in Hungary between 2010 and 2020 and to shed light on some aspects and their interrelations. To identify spatial and social processes, we formulated research questions and analyzed these questions with spatial econometrical methods, like Eigenvector Spatial Filtering.
As a result, a decrease in spatial income inequalities between territorial units and, simultaneously, an increase in income disparities within territorial units can be observed that are geographically differentiated. The study concludes that there is an important spatial difference in the performance of the LAU1 units of Hungary regarding the regional revealed competitiveness, which was influenced by various economic, spatial, and social factors between 2010 and 2020.
Acknowledgment:"GYÖRGY VIDA HAS CONTRIBUTION SUPPORTED BY THE ÚNKP-22-4-136 NEW NATIONAL EXCELLENCE PROGRAM OF THE MINISTRY FOR CULTURE AND INNOVATION FROM THE SOURCE OF THE NATIONAL RESEARCH, DEVELOPMENT AND INNOVATION FUND.”
Consequently, the study aims to examine the geographical patterns of competitiveness and income disparities in Hungary between 2010 and 2020 and to shed light on some aspects and their interrelations. To identify spatial and social processes, we formulated research questions and analyzed these questions with spatial econometrical methods, like Eigenvector Spatial Filtering.
As a result, a decrease in spatial income inequalities between territorial units and, simultaneously, an increase in income disparities within territorial units can be observed that are geographically differentiated. The study concludes that there is an important spatial difference in the performance of the LAU1 units of Hungary regarding the regional revealed competitiveness, which was influenced by various economic, spatial, and social factors between 2010 and 2020.
Acknowledgment:"GYÖRGY VIDA HAS CONTRIBUTION SUPPORTED BY THE ÚNKP-22-4-136 NEW NATIONAL EXCELLENCE PROGRAM OF THE MINISTRY FOR CULTURE AND INNOVATION FROM THE SOURCE OF THE NATIONAL RESEARCH, DEVELOPMENT AND INNOVATION FUND.”
Dr. Alberto Tidu
Post-Doc Researcher
Università di Cagliari - CRENoS
Measuring spatial dispersion: an experimental test on the M-index
Author(s) - Presenters are indicated with (p)
Alberto Tidu (p), Stefano Usai, Frederick Guy
Discussant for this paper
Izabella Szakálné Kanó
Abstract
We assess the viability of a geographic approximation aimed to reduce the computational intensity necessary to measure spatial agglomeration with Marcon & Puech’s (2017) M index. Indeed, despite representing a potentially very accurate way of measuring spatial distribution, M has not been sufficiently exploited so far because its computation needs crossing every point (i.e. firms, plants) with each other within the area under analysis: such a figure grows to unmanageable levels when the area is larger than a neighborhood or when every industry is taken into account. Consequently, applications of M have been exclusively experimental and circumscribed to very limited areas or to a handful of sectors. This seems much regrettable since M provides many advantages compared to more conventional measures of spatial distribution and also to other distance measures.
In order to verify whether a slight geographic approximation is tolerable – which would be consistent with Marcon & Puech’s assumption that “cumulative functions are insensitive to errors at smaller scales than the distance they consider” - we compute both actual M (with no approximation whatsoever) and approximate M for every industry in Sardinia. Our aim is to compare the results obtained when plants are located exactly where they are with those obtained when plants’ positions are approximated to the centroid of the municipality where they are located.
We rely on a comprehensive dataset that allows us to identify the location, the industry and the number of employees for every single plant, and not only for entire firms. Our dataset’s scope is not restricted to manufacturing, as it is often the case, but covers every area of activity, ranging from construction to transports and from retailers to other service industries. Moreover, we did not consider distance between approximated positions as the crow flies, but we relied on actual street distance and travel time between them: in the frequent case of orographically dishomogenous territories, it might be the case that such a measurement reflects the actual distance between the approximated locations of establishments even more accurately than the theoretical flying distance between actual locations.
If our approximation in the location of plants is positively outweighed by the great accuracy of M in operationalizing detailed geographic and economic information, then such an index could really be exploited for assessing agglomeration and dispersion patterns across space and along time, especially when much information is available, as it is ever more often the case.
In order to verify whether a slight geographic approximation is tolerable – which would be consistent with Marcon & Puech’s assumption that “cumulative functions are insensitive to errors at smaller scales than the distance they consider” - we compute both actual M (with no approximation whatsoever) and approximate M for every industry in Sardinia. Our aim is to compare the results obtained when plants are located exactly where they are with those obtained when plants’ positions are approximated to the centroid of the municipality where they are located.
We rely on a comprehensive dataset that allows us to identify the location, the industry and the number of employees for every single plant, and not only for entire firms. Our dataset’s scope is not restricted to manufacturing, as it is often the case, but covers every area of activity, ranging from construction to transports and from retailers to other service industries. Moreover, we did not consider distance between approximated positions as the crow flies, but we relied on actual street distance and travel time between them: in the frequent case of orographically dishomogenous territories, it might be the case that such a measurement reflects the actual distance between the approximated locations of establishments even more accurately than the theoretical flying distance between actual locations.
If our approximation in the location of plants is positively outweighed by the great accuracy of M in operationalizing detailed geographic and economic information, then such an index could really be exploited for assessing agglomeration and dispersion patterns across space and along time, especially when much information is available, as it is ever more often the case.
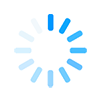