Alicante-G42 Big Data and Regional Science
Tracks
Refereed/Ordinary Session
Thursday, August 31, 2023 |
14:30 - 16:15 |
1-B13 |
Details
Chair: Gunther Maier
Speaker
Dr. Olle Järv
Senior Researcher
University Of Helsinki
Understanding cross-border regions from the mobility of people: New insights from Big Data
Author(s) - Presenters are indicated with (p)
Olle Järv (p), Håvard Wallin Aagesen, Ate Poorthuis
Discussant for this paper
Gunther Maier
Abstract
One paramount priority for the EU is to enhance growth and cohesion in the internal border regions through cross-border cooperation and governance. To understand how cohesive policies and effective planning and development enhance cross-border integration, we need information from the perspective of people. In the EU, people cross borders for various reasons – migration, tourism, work and education, meeting family and friends. We also witness increasingly more people whose daily lives are not confined to a fixed territory of one country such as cross-border commuters and people with multi-local living lifestyles between different countries. These interactions and mobilities crossing country borders not only affect individuals’ social connectedness and integration (e.g. social networks and place attachment) in border region, but also contribute to the (re)production of functional cross-border regions.
Regardless of its growing importance, however, little attention has been paid to cross-border practices of (local) people beyond migration and tourism. How, where, when and why habitual and recurring mobilities across country borders take place? What kind of temporal rhythms and trends these cross-border practices have over time? How cross-border practices of people are affected by external factors? How to evaluate the impact of institutional instruments (e.g. the ERDF) on the daily lives of local people in border region? For these questions we lack appropriate data source – register and census data can’t capture the dynamic nature of border mobility, and large-scale surveys are time and resource consuming capturing only one point of time.
We propose that inherently transnational big data sources like social media can provide much needed new insights into cross-border mobilities. Stemming from literature showing that social media reveals mobility of people, we consider human mobility as a tool to understand society – it indicates social interactions that form functional systems like border regions.
We show in case of several case studies in Europe as the proof-of-concept how openly available Twitter data can reveal spatial distribution of cross-border mobility flows, its temporal rhythms and how it varies by mobility type. We demonstrate how functional border regions can be delineated from mobility flows and compare with official Interreg regions. We discuss the feasibility of our approach to monitor development projects, evaluate cooperation and governance actions of border regions from the perspective of (local) people. Finally, we address future avenues in enhancing our conceptual framework and methodology.
Regardless of its growing importance, however, little attention has been paid to cross-border practices of (local) people beyond migration and tourism. How, where, when and why habitual and recurring mobilities across country borders take place? What kind of temporal rhythms and trends these cross-border practices have over time? How cross-border practices of people are affected by external factors? How to evaluate the impact of institutional instruments (e.g. the ERDF) on the daily lives of local people in border region? For these questions we lack appropriate data source – register and census data can’t capture the dynamic nature of border mobility, and large-scale surveys are time and resource consuming capturing only one point of time.
We propose that inherently transnational big data sources like social media can provide much needed new insights into cross-border mobilities. Stemming from literature showing that social media reveals mobility of people, we consider human mobility as a tool to understand society – it indicates social interactions that form functional systems like border regions.
We show in case of several case studies in Europe as the proof-of-concept how openly available Twitter data can reveal spatial distribution of cross-border mobility flows, its temporal rhythms and how it varies by mobility type. We demonstrate how functional border regions can be delineated from mobility flows and compare with official Interreg regions. We discuss the feasibility of our approach to monitor development projects, evaluate cooperation and governance actions of border regions from the perspective of (local) people. Finally, we address future avenues in enhancing our conceptual framework and methodology.
Mr Alexandre Banquet
Junior Researcher
OECD Centre for Entrepreneurship, SMEs, Regions and Cities
Monitoring land use in cities using satellite imagery and deep learning
Author(s) - Presenters are indicated with (p)
Alexandre Banquet (p), Paul Delbouve, Michiel Daams, Paolo Veneri
Discussant for this paper
Olle Järv
Abstract
Over time, cities expand their physical footprint on land and new cities emerge. The shape of the built environment can affect several domains which are policy relevant, such as carbon emissions, housing affordability, infrastructure costs, resource consumption, and access to services. This study lays a methodological basis for the monitoring and consistent comparison of land use across OECD metropolitan areas. An advanced form of deep learning-based image segmentation framework, namely the U-Net model, is trained on the Copernicus Urban Atlas to classify land use and land cover in EC-ESA Sentinel-1 and -2 satellite imagery. The paper shows that this model enables to predict land-use in newly seen satellite images with high accuracy. It is also able to disentangle mostly residential areas, from commercial and industrial areas. This work complements conventional statistical data by monitoring large surfaces of land efficiently and in near real-time. In specific, following the availability of detailed data for model training, built-up areas in residential or business-related use are mapped and analysed for 687 European metropolitan areas, as a case application. This paper also explores potential applications in OECD metropolitan areas located beyond Europe, as well as for change detection to spot recent urban expansion’s speed and shape signals.
Dr. Hyelim Kim
Ph.D. student
Gyeongsang National University
Prediction of crime occurrence probability using machine learning and selection of CCTV installation locations
Author(s) - Presenters are indicated with (p)
Hyelim Kim (p), TaeHeon Moon, SunYoung Heo
Discussant for this paper
Alexandre Banquet
Abstract
Our city is exposed to crime, and for the safety of citizens, it is necessary to analyze the urban space and come up with efficient countermeasures. One representative measure for crime prevention is the installation of CCTV in crime-prone locations. This study is divided into two stages. The first stage involves predicting areas with high crime rates in Sacheon, Korea, using crime data such as time and place, spatial data, and meteorological data processed in units of spatial 200mx200m cells. Machine learning is then used to predict and visualize areas with a high probability of crime on a cell-by-cell basis. Machine learning was applied using various algorithms, such as regression and decision trees, with training and test data sets in ratios of 7:3, 8:2, and 9:1. The prediction accuracy was identified by RMSE (Root Mean Squared Error), and the model with the lowest RMSE value was selected as the optimal model. In the second stage, blind spots in areas with high crime rates are identified, taking into account the current location and angle of view of existing CCTV cameras according to the height of the building. We assess the adequacy of the existing CCTV locations and identify additional installation points to maximize the effectiveness of crime prevention. Currently, Sacheon City is discussing the installation of CCTV based on the results of this study.
Prof. Gunther Maier
Full Professor
Modul University Vienna
Where is ERSA’s journal REGION positioned in the Regional Science publishing world?
Author(s) - Presenters are indicated with (p)
Gunther Maier (p), Tom Brökel, Francisco Rowe
Discussant for this paper
Hyelim Kim
Abstract
The journal of ERSA, REGION, is fairly unique among Regional Science journals as it publishes free for readers and free for authors. Since most other journals in the discipline are published by one of the big publishing companies and charge article processing fees for Open Access publication, REGION also represents an alternative model of scholarly publishing.
One can expect that all these differences will lead to differences in the position of REGION as compared to other journals in the discipline. In this paper, we will analyze the position of REGION in the community, try to answer the question of whether differences exist compared to other Regional Science journals and describe those differences. We will use the full range of data we have available for REGION, i.e., data on submissions, publications, downloads, and citations (both outgoing and incoming). Where possible, we will compare the results to selected other regional science journals.
The paper will use methods of scientometrics, network analysis, text mining, and clustering in order to extract the necessary information about REGION. Since comparable data are usually not available for journals published by commercial publishing companies, comparison will be limited to a smaller range of indicators.
One can expect that all these differences will lead to differences in the position of REGION as compared to other journals in the discipline. In this paper, we will analyze the position of REGION in the community, try to answer the question of whether differences exist compared to other Regional Science journals and describe those differences. We will use the full range of data we have available for REGION, i.e., data on submissions, publications, downloads, and citations (both outgoing and incoming). Where possible, we will compare the results to selected other regional science journals.
The paper will use methods of scientometrics, network analysis, text mining, and clustering in order to extract the necessary information about REGION. Since comparable data are usually not available for journals published by commercial publishing companies, comparison will be limited to a smaller range of indicators.
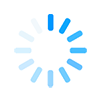