Alicante-S27-S1 Applications of Advanced and Innovative Methods in Regional Science
Tracks
Special Session
Friday, September 1, 2023 |
9:00 - 10:30 |
1-C13 |
Details
Chair: Katarzyna Kopczewska - University of Warsaw, Poland, Emmanouil Tranos - University of Bristol, England
Speaker
Prof. Manfred M. Fischer
Full Professor
Vienna University of Economics and Business
Opioid Mortality in the US: Quantifying the Impact of Key Determinants Using a Spatial Panel Data Approach
Author(s) - Presenters are indicated with (p)
Manfred M. Fischer (p), Sucharita Gopal
Discussant for this paper
Katarzyna Kopczewska
Abstract
This paper employs a spatial Durbin panel data model, an extension of the cross-sectional spatial Durbin model to a panel data framework, to quantify the impact of a set of sociodemographic and socioeconomic factors that influence opioid-related mortality in the US. The empirical model uses a pool of 49 US states over six years from 2014 to 2019, and a nearest neighbor matrix that represents the topological structure between the states. Calculation of direct (own-state) and indirect (cross-state spillovers) effects estimates is based on Bayesian estimation and inference reflecting a proper interpretation of the marginal effects for the model that involves spatial lags of the dependent and independent variables.
The study provides evidence that opioid mortality depends not only on the characteristics of the state itself (direct effects), but also on those of nearby states (indirect effects). Direct effects are important, but externalities (spatial spillovers) are more important. The sociodemographic structure (age and race) of a state is important whereas economic distress of a state is less so, as indicated by the total impact estimates. The methodology and the research findings provide a useful template for future empirical work using other geographic locations or shifting interest to other epidemics.
The study provides evidence that opioid mortality depends not only on the characteristics of the state itself (direct effects), but also on those of nearby states (indirect effects). Direct effects are important, but externalities (spatial spillovers) are more important. The sociodemographic structure (age and race) of a state is important whereas economic distress of a state is less so, as indicated by the total impact estimates. The methodology and the research findings provide a useful template for future empirical work using other geographic locations or shifting interest to other epidemics.
Prof. Jeffrey Zax
Full Professor
University of Colorado Boulder
Why incomes should never be adjusted for cross-sectional variations in prices
Author(s) - Presenters are indicated with (p)
Jeffrey Zax (p)
Discussant for this paper
Manfred M. Fischer
Abstract
Cross-sectional comparisons of welfare are interesting in many contexts. These comparisons are usually estimated through cross-sectional comparisons of incomes. When these comparisons are between agents who reside in different physical locations, agents may differ in the prices that they face as well as in the incomes that they receive. This paper describes the proper treatment of cross-sectional price variations in welfare comparisons. When factors and final goods are mobile, or when only final goods are mobile but factor price equalization occurs, prices can differ inter-regionally only for non-traded goods. Any such differences represent capitalization of unpriced amenities. Therefore, higher observed prices are indicative of a region that is rich in amenities rather than expensive for consumption. Standard “cost-of-living” adjustments are systematically biased because they underestimate utility in these regions and overstate utility in regions that are poor in unpriced amenities. In contrast, unadjusted incomes are not biased under these mobility assumptions. If inter-regional mobility is further constrained, the relationship between inter-regional price differentials and inter-regional welfare differentials is fundamentally ambiguous. In these contexts, standard “cost-of-living” adjustments are essentially uninterpretable
Prof. Paul Elhorst
Full Professor
Rijksuniversiteit Groningen
The distance decay effect and spatial reach of economic growth spillovers
Author(s) - Presenters are indicated with (p)
Paul Elhorst (p)
Discussant for this paper
Jeffrey Zax
Abstract
This paper presents a methodology to estimate the distance decay effect and spatial reach of spillover effects in a spatial Durbin (SD) model. Building on concepts about spatial autocorrelation developed by Getis (2010), we adopt a distance-based negative exponential spatial weight matrix and parameterize it by a distance decay parameter that is different for each spatial lag. The methodology is illustrated based on a spatially augmented neoclassical growth framework and estimated using data of 266 NUTS-2 regions in the EU over the period 2000-2018. We find that the distance decay parameters range from 0.12 to 8.62 and that the model based on one common spatial weight matrix for all spatial lags in the SD model is too restrictive. We also document the extent to which a change in each growth determinant spills over to other regions in terms of distance, sign, magnitude and significance level.
Prof. Katarzyna Kopczewska
Associate Professor
University of Warsaw
Spatial switching regimes regression: density-based cluster approach to deal with spatial heterogeneity
Author(s) - Presenters are indicated with (p)
Katarzyna Kopczewska (p), Ewa Dobrowolska
Discussant for this paper
Paul Elhorst
Abstract
Spatial micro-econometric modelling often neglects the density of point data, which can generate spatial heterogeneity between high- and low-density areas. This can cause unjustified insignificance of regression coefficients and lead to wrong conclusions on relations between variables. In this paper, we propose the density-based spatial switching regimes regression, a semi-local regression approach that allows for obtaining group-diversified regression intercepts and slopes. We use the DBSCAN algorithm to detect spatially-continuous areas with similar densities of analysed points and conduct multi-group regressions and comparisons. Our model offers a solution between Geographically Weighted Regression (GWR) and global models and addresses the spatial heterogeneity resulting from diversified density. We evidence the importance of density-based modelling and demonstrate the efficiency of our approach compared to old competitive models. Our findings have important research and policy implications for spatial micro-econometric modelling.
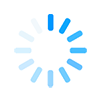