Alicante-YS01 People-based Development and Policies - EPAINOS
Thursday, August 31, 2023 |
11:00 - 13:00 |
0-C01 |
Details
Chair & Discussant: Raquel Ortega-Argilles
Speaker
Mr Alper Acar
Ph.D. Student
Université de Bourgogne
Where and how to locate a new population? Inverse location models in response to rural development challenges.
Author(s) - Presenters are indicated with (p)
Alper Acar (p)
Abstract
The growing need for housing in France is a challenge under several constraints. Legally, construction is subject to the Local Urban Plan (“Plan Local d’Urbanisme”) and the law of zero net artificialization (“Zero Artificialisation Nette”) created to fight against urban sprawl. Theoretically, building new housing means introducing a new population into the area. This population must then have access to the best possible range of facilities (healthcare, for example). The traditional location models deal with finding the optimal location of new facilities respect to given criteria such as minimizing time, cost or distanced between the given client points and facilities. The inverse and reverse models try to modify the parameters of the problem, such that one or several points becomes optimal, or according to a budget, respectively. This paper focuses on modifying the users’ weights (locating a new population) by introducing two models. Firstly, The New Population Problem (NPP) aims to locate an additional population under the same objectives by maximizing entropy. Secondly, The Regret Minimization Problem aims to minimize the regret, i.e., the difference of the value of the median objective function with the new and the current users’ weights. An application for each model based on real data in Dijon Métropole (France) is proposed. We assume the new weights to be optimal according to the location of health facilities. Results show that the new population is located near the facilities and are relevant with the Local Urban Plan.
Dr. Paolo Bottero
Post-Doc Researcher
Gran Sasso Science Institute
The reconstruction of L’Aquila in people’s emotions. Using Artificial Intelligence to retrace sentiments towards the long-term post-disaster recovery process
Author(s) - Presenters are indicated with (p)
Paolo Bottero (p)
Abstract
In recent years, the study of sentiments expressed on social media in disaster scenarios has increased. The role of social platforms in disaster management has traditionally been focused on the crucial function that microblogs take for real-time data or in the very early stages of recovery. Relatively less attention has been devoted to the sentiment expressed in the long term. To understand the long-run effects of a disaster on public emotions, this paper examines the sentiments towards the city of L’Aquila and its recovery process after the 2009 earthquake using Twitter data collected for more than a decade. The analysis is performed using a Robustly Bidirectional Encoder Representations from Transformers (RoBERTa) classification model that categorises 53610 tweets by sentiment (positive or negative) and emotion (anger, fear, joy, sadness). To disentangle the causes behind these sentiments, a text analysis is performed in order to extract the ten most frequent words that characterise tweets for each emotion. The study shows that the sadness and fear of the immediate post-disaster are replaced with a constant increase in public anger in the first five years of stagnation of the reconstruction process. Anger is exacerbated by factors such as excessive politicisation, the controversial communication of the early post-disaster, corruption, and inadequate early emergency policies. In the midterm, the start of the reconstruction and the investments in cultural events partly mitigate anger. After ten years, with the process projected to its end, the public opinion on L’Aquila reconstruction becomes constant, without significant changes in the proportion of anger, fear, joy, or sadness, with the latter remaining predominant in the few reconstruction-related tweets. In addition to the analysis of the post-disaster dynamics and the effect of reconstruction policies, this paper highlights the role that AI and sentiment analysis can play in reconstructing the phases of long-term post-disaster management.
Mr Eric Myran Wee
Ph.D. Student
Norwegian University of Science and Technology
Agglomeration effects of immigrants and natives: Heterogeneity based on country of birth
Author(s) - Presenters are indicated with (p)
Eric Myran Wee (p)
Abstract
I investigate the urban wage premium for natives and groups of immigrants based on country of birth. The findings presented here add to the scarce literature on the agglomeration effects of immigrants. Using rich administrative data for Norway from 2008-2019 with information on labor participation back to 1993, I find that immigrants from low-income countries lag behind natives and immigrants from high-income countries from a lack of an immediate large-city earnings premium. The gap is increasing over time as immigrants from low-income countries have a lower return to work experience accumulated in cities than natives and immigrants from high-income countries. However, when separating the sample based on educational attainment, the results suggest that the lower return to urban experience for immigrants from low-income countries is mainly driven by primary educated individuals. I find that the return to acquired urban experience is higher for individuals with higher initial ability, as measured with worker fixed effects.
Chair & Discussant
Raquel Ortega-Argiles
Full Professor
University of Manchester
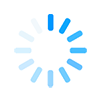