Pecs-S59-S3 Spatial Coronametrics: New Tools in Regional Science for Quantifying the Spatial Dimensions of Pandemics
Tracks
Day 3
Wednesday, August 24, 2022 |
16:00 - 17:30 |
A308 |
Details
Chair: Jaewon Lim
Speaker
Prof. Danny Czamanski
Full Professor
Ruppin Academic Center
Land value dynamics and the spatial evolution of cities following COVID 19 using big data analytics
Author(s) - Presenters are indicated with (p)
Danny Czamanski (p), Dani Broitman (p), Erez Buda
Discussant for this paper
Sarah Low
Abstract
In this paper we present results of a land-use forecasting model that we calibrated with vast geo-referenced data of a major metropolitan area. Each land parcel includes information concerning regulations indicating permitted land-uses as well as the certain characteristics of existing buildings. Data concerning all real estate transactions include information about the assets and the price of the exchanges. Based on these data we estimated the spatial dynamics of land values in the metropolitan area over time and identified locations experiencing development pressures. This analysis allows us to forecast plausible futures of the urban spatial configuration. Taking the approach one step further, we propose simulations motivated by the natural experiment of COVID 19. We assumed that part of the behavioral changes observed during the pandemic will endure. The resulting simulations provide forecasts of the future spatial structure of the metropolitan area. Comparing the actual and the forecasted scenarios we interpret the spatial dynamics of the city as they would be if a business-as-usual-pre-Covid-19 scenario is realized, and possible trend changes if the impact of the pandemic is long lasting.
Prof. Laurie Schintler
Associate Professor
George Mason University
Genomic Surveillance Data for Regional COVID-19 Machine Learning Predictive Analytics: What Do We Gain and What Works Best?
Discussant for this paper
Danny Czamanski
Abstract
The complexities of the COVID-19 pandemic create significant challenges for regional forecasting of the spread and impact of the novel coronavirus. One issue is that SARS-VoV-2 is continually evolving, changing its genetic code as it travels from one place to another and over time. Moreover, multiple variants are circulating in the population at any given point. Complicating matters further is that some strains are more virulent or easily transmissible than others; others are resistant to prevailing approaches to prevention and treatment. This paper examines how and to what extent genomic surveillance data can improve the accuracy of regional COVID machine learning predictive analytics models. However, this approach poses some analytical and computational challenges. First, genomic sequencing data is a convenience sample. Accordingly, there are substantial regional and temporal variations in the number and percent of sequences collected and shared. Second, it is a high-dimensional data problem. Thirdly, it may be necessary to account for spatio-temporal autocorrelation and heterogeneity. In light of these issues, we explore the relative performance of different statistical machine learning algorithms (e.g., SVM, Ridge/Lasso regression, regression tree, etc.) for regional COVID forecasting. We focus on regions in the United States for the experiments, drawing upon genomics sequencing data from the GISAID and related repositories. The paper will conclude with some lessons for regional epidemiological modeling, as well as policy implications and recommendations.
Prof. Neil Reid
Full Professor
University Of Toledo
Meeting the Challenge of COVID-19: How American Craft Breweries Responded
Author(s) - Presenters are indicated with (p)
Neil Reid (p), Margaret Gripshover, Thomas Bell
Discussant for this paper
Laurie Schintler
Abstract
The appearance and subsequent diffusion of COVID-19 forced many bars and restaurants across the United States to close their doors and discontinue normal business practices. This included over 8,000 craft breweries. As a result of COVID-19 directives, craft breweries were restricted to curbside pick-up and home delivery of the beer they produced, forcing them to rethink and redesign an important part of their business model. The primary purpose of this presentation is to assess how craft breweries responded to the restrictions placed upon them. A historical review of how the 1918-19 influenza pandemic impacted the brewing industry in the United States is also provided. Similarities between how COVID-19 and the 1918-19 influenza pandemics impacted their period’s respective brewing industries are identified.
Prof. Sarah Low
Associate Professor
University Of Missouri
Has COVID-19 made rural areas more attractive places to live? Survey evidence from a U.S. region
Author(s) - Presenters are indicated with (p)
Sarah Low (p), Mallory Rahe, Andrew Van Leuven
Abstract
COVID-19 disruptions encouraged some rural regions to think about proactively attracting newly footloose residents---but would the pandemic make rural areas seem more attractive to potential return migrants? Using econometric analysis of survey data, we do not find evidence of this. To natives who had left the study region, attitudes about living in rural areas during COVID were lower on average than for those who stayed. We find, however, that owning a business and a stronger sense of belonging are both associated with positive attitudinal shifts towards rural living, which has practical implications for rural migration policy.
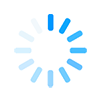