Pecs-G14-O2 Spatial Econometrics
Tracks
Day 4
Thursday, August 25, 2022 |
16:00 - 17:30 |
B016 |
Details
Chair: Philipp Piribauer
Speaker
Dr. Dávid Sümeghy
Junior Researcher
University of Pécs
Validating the impact of spatial spillovers on party preference
Author(s) - Presenters are indicated with (p)
Dávid Sümeghy (p)
Discussant for this paper
Philipp Piribauer
Abstract
Research on the growing support of radical right parties cannot ignore the role of spatial, contextual effects and geographical proximity. The most commonly mentioned spatial factor in the literature is the halo effect, which suggests that a radical right party is more likely to perform well in a homogeneous electoral district, surrounded by ethnically diverse areas. In this paper, I model the 2018 election results of the Sweden Democrats at the level of the 6008 Swedish electoral districts. I define the halo effect as a spillover effect of the ethnic structure of the neighbouring areas using different spatial weight matrices. The spatial models augmented with Durbin components (Spatial Durbin Model and Spatial Durbin Error model, chosen after the Lagrange Multiplier tests) show a better fit and indicate a spillover effect of the level of education, aggregate employment and median wealth in addition to the contextual role of ethnic diversity. Compared to the map of residuals from the OLS model, spatial modelling resulted in particularly significant improvements in some of Skåne County's electoral districts. To validate the results of the spatial modelling, I will carry out a hybrid mail-online survey in the electoral districts with significant improvements regarding the residuals. The survey will be conducted during May or June. The questionnaire survey will aim to verify the role of spatial spillover effects and to explore possible causes (political contamination of social interactions, copy-cat behaviour, the role of media, commuting) that may trigger them.
Mr Michael Wögerer
Ph.D. Student
International Institute for Applied Systems Analysis
Coefficient accuracy in regression models with spatially varying coefficients
Author(s) - Presenters are indicated with (p)
Michael Wögerer (p)
Discussant for this paper
Dávid Sümeghy
Abstract
The paper examines the effect of multicollinearity in two state of the art models with spatially varying coefficients. The two models under scrutiny, geographically weighted regressions (GWR) and spatially varying coefficient processes (SVCP), alleviate the assumption of constant parameters over the whole observed geographic area and allow for regional variations in the estimated regression coefficients. However, such models suffer from inefficiency and identification issues with rising levels of collinearity in the explanatory variables, resulting in biased an inconsistent marginal inference. This paper on the one hand explores various possible scenarios to evaluate coefficient accuracy in regression models with spatially varying coefficients by means of a simulation study with varying levels of correlation among the explanatory variables, different values of disturbance in the models, and different degrees of spatial dependence. On the other hand, using recent advances in Bayesian computing, the paper proposes solutions to reduce the level of variable collinearity and, therefore, improve coefficient accuracy. Specifically, variable selection techniques and shrinkage priors are employed to tackle overparameterization which is the most common culprit for collinearity. The results highlight that variable selection and shrinkage are indeed easy extensions and can substantially enhance parameter accuracy. The paper also showcases the performance of the proposed modeling framework in estimating urban expansion in European NUTS2 regions using detailed land-use information. The application indicates that allowing for spatial variations in the regression coefficients plays a key role in understanding observed land-sealing in European regions.
Ms Ioanna Tziolas
Ph.D. Student
University of Groningen
Trade, Gravity and Cross-Sectional Dependence
Author(s) - Presenters are indicated with (p)
Paul Elhorst, Petros Milionis, Ioanna Tziolas (p)
Discussant for this paper
Michael Wögerer
Abstract
Trade flows tend to exhibit a high degree of cross-sectional dependence (CSD) because they vary systematically over space. This paper first documents that the standard estimation of the log-linearized gravity equation with higher-order fixed effects does not fully account for CSD, as indicated by the CD test developed by Pesaran (2015, Econometric Reviews) and the exponent α developed by Bailey et al. (2016, Journal of Applied Econometrics). We document this pattern with two panel data sets: one at the country level (306 country pairs) and one at the regional level (70756 regional pairs).
We then propose two approaches to account for the observed CSD in trade flows within a common estimation framework. The first approach follows the literature on dynamic factor models and includes in the specification of the gravity equation unobserved heterogeneous global and local factors instead of time-varying exporter and importer fixed effects. The global factors are approached by the cross-sectional average of the trade flows in each time period and the local factors by the time-average of trade flows of each exporter and importer. The second approach follows the spatial econometric literature and introduces spatial lags of the dependent variable or the error term in the gravity equation. We specifically consider (i) spatial autoregressive (SAR) specifications, (ii) higher-order SAR specifications, and (iii) spatial autoregressive moving-average (SARMA) specifications, which assume that trade flows between two economies depend mostly on the trade flows of their neighbors. The two approaches differ in the extent to which they consider CSD to be of global or of local nature (strong versus weak CSD). We further combine the two approaches to estimate spatial econometric models that include global and local factors.
The results indicate that both approaches can be useful in mitigating the problem of CSD. Yet, the relative merits of the two approaches differ across the two data sets. When using country data, we find that global and local factors are sufficient in filtering out CSD while the inclusion of spatial lags does not seem necessary. When using regional data, the degree of CSD is found to be more localized to be captured by global and common factors. Hence, in this case, the inclusion of spatial lags is more important. Overall our findings suggest that the right approach to deal with CSD in gravity estimation should be based on the nature of data under consideration.
We then propose two approaches to account for the observed CSD in trade flows within a common estimation framework. The first approach follows the literature on dynamic factor models and includes in the specification of the gravity equation unobserved heterogeneous global and local factors instead of time-varying exporter and importer fixed effects. The global factors are approached by the cross-sectional average of the trade flows in each time period and the local factors by the time-average of trade flows of each exporter and importer. The second approach follows the spatial econometric literature and introduces spatial lags of the dependent variable or the error term in the gravity equation. We specifically consider (i) spatial autoregressive (SAR) specifications, (ii) higher-order SAR specifications, and (iii) spatial autoregressive moving-average (SARMA) specifications, which assume that trade flows between two economies depend mostly on the trade flows of their neighbors. The two approaches differ in the extent to which they consider CSD to be of global or of local nature (strong versus weak CSD). We further combine the two approaches to estimate spatial econometric models that include global and local factors.
The results indicate that both approaches can be useful in mitigating the problem of CSD. Yet, the relative merits of the two approaches differ across the two data sets. When using country data, we find that global and local factors are sufficient in filtering out CSD while the inclusion of spatial lags does not seem necessary. When using regional data, the degree of CSD is found to be more localized to be captured by global and common factors. Hence, in this case, the inclusion of spatial lags is more important. Overall our findings suggest that the right approach to deal with CSD in gravity estimation should be based on the nature of data under consideration.
Dr. Philipp Piribauer
Post-Doc Researcher
Austrian Institute Of Economic Research (wifo)
A Bayesian approach for estimation of weight matrices in spatial autoregressive models
Author(s) - Presenters are indicated with (p)
Tamás Krisztin, Philipp Piribauer (p)
Discussant for this paper
Ioanna Tziolas
Abstract
In the spatial econometric literature, approaches that directly target estimation of spatial weight matrices are scarce. Our paper proposes a novel and flexible Bayesian approach for direct estimation of spatial weight matrices. Our definition of spatial weight matrices in this study fulfils the typical assumptions employed in the vast majority of spatial econometric literature. The resulting spatial weight matrices are assumed non-negative and requirements to identification of the parameters can be easily implemented. Albeit we focus on row-standardized spatial weights, spatial weights without row-standardization are also implementable. To tackle potential over-parameterization problems a priori, the proposed approach also allows a direct implementation of a symmetric neighbourhood structure in a straightforward way. The resulting flexibility comes at the price that we focus on spatial adjacency matrices which are binary (prior to row-standardization). However, this assumption is widely used in the spatial econometric literature (e. g. spatial weight matrices based on concepts of contiguity, distance-band, or 𝑘-nearest neighbours).
Monte Carlo simulations show that our approach is particularly useful in spatial panels where T is small relative to N, which is a rather common characteristic of regional economic datasets. The virtues of the proposed approach are moreover demonstrated using real data on the global outbreak of the COVID-19 pandemic.
Monte Carlo simulations show that our approach is particularly useful in spatial panels where T is small relative to N, which is a rather common characteristic of regional economic datasets. The virtues of the proposed approach are moreover demonstrated using real data on the global outbreak of the COVID-19 pandemic.
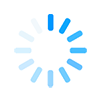