G03-O4 Regional competitiveness, innovation, and productivity
Tracks
Ordinary Session
Thursday, August 30, 2018 |
9:00 AM - 10:30 AM |
WGB_302 |
Details
Chair: Bettina Becker
Speaker
Prof. Raphael Bar-El
Full Professor
Ben Gurion University
Innovation explained: an analytical model
Author(s) - Presenters are indicated with (p)
Raphael Bar-El (p)
Abstract
A huge variety of variables are used by different organizations in order to evaluate the level of innovation in a region or in a country, resulting in diverse rankings. The intention in this paper is to propose a simple analytical model in which the various measures used for the evaluation of innovation are classified into four main groups, and explanatory relations between them are tested.
Following the distinction made by the GII (global innovation index) between innovation inputs and outputs, we first clearly discern between two groups of outputs: “knowledge creation” and “innovation creation”. The first represents the creation of pure knowledge with no immediate macro-economic or social benefit (such as the creation of patents or of new business models), and the second represents the use of knowledge for the creation of innovative activities (such as the manufacturing of high-tech products). All output variables are defined as endogenous variables.
Input variables are also classified in two groups: “innovation initiators” and “enabling factors”, both defined as exogenous variables. “Knowledge creation” is a function of “innovation initiators” (such as R&D activity), while “innovation creation is a function of both “knowledge creation” and “enabling factors” (such as the availability or skilled labor force).
We estimate through regression analysis the links between main inputs and outputs. The statistical analysis is based on the GII data for 2017, for 137 countries.
The results show main determinants of knowledge creation (R&D, education, linkages, ICTs). Innovation practical outputs depend naturally on the creation of knowledge, but are heavily conditioned by the ability of firms to absorb innovation, both internal and external, the access to relevant skills, and the technological and business environment. The “efficiency index” as shown by GII can certainly be a result of a gap between the creation of knowledge and the ability of the economy to transform such knowledge into practical innovation activities.
An important conclusion is that innovation has different facets, each responding differently to different factors. Economies or regions with certain “enabling factors” may be more responsive to non-technological innovation, while certain types of knowledge creation may be more appropriate for open economies.
Following the distinction made by the GII (global innovation index) between innovation inputs and outputs, we first clearly discern between two groups of outputs: “knowledge creation” and “innovation creation”. The first represents the creation of pure knowledge with no immediate macro-economic or social benefit (such as the creation of patents or of new business models), and the second represents the use of knowledge for the creation of innovative activities (such as the manufacturing of high-tech products). All output variables are defined as endogenous variables.
Input variables are also classified in two groups: “innovation initiators” and “enabling factors”, both defined as exogenous variables. “Knowledge creation” is a function of “innovation initiators” (such as R&D activity), while “innovation creation is a function of both “knowledge creation” and “enabling factors” (such as the availability or skilled labor force).
We estimate through regression analysis the links between main inputs and outputs. The statistical analysis is based on the GII data for 2017, for 137 countries.
The results show main determinants of knowledge creation (R&D, education, linkages, ICTs). Innovation practical outputs depend naturally on the creation of knowledge, but are heavily conditioned by the ability of firms to absorb innovation, both internal and external, the access to relevant skills, and the technological and business environment. The “efficiency index” as shown by GII can certainly be a result of a gap between the creation of knowledge and the ability of the economy to transform such knowledge into practical innovation activities.
An important conclusion is that innovation has different facets, each responding differently to different factors. Economies or regions with certain “enabling factors” may be more responsive to non-technological innovation, while certain types of knowledge creation may be more appropriate for open economies.
Dr. Achim Ahrens
Post. Doc Researcher
Economic And Social Research Institute
A Regional Perspective on Industrial Production in Ireland: A Spatial Stochastic Frontier Approach
Author(s) - Presenters are indicated with (p)
Achim Ahrens (p), Edgar Morgenroth Morgenroth
Abstract
Ireland, as most countries, is characterised by regional disparities. Economic activity and prosperity are concentrated in a few regional clusters, in particular Cork and Dublin. In this study, we contribute to the literature on regional disparities in Ireland by considering a county-level panel data set of industrial output from the Irish Census of Industrial Production covering 1979-2009. We employ efficiency analysis to identify counties which conditional on capital and labour inputs exhibit low output levels.
Spatial or cross-sectional dependence is expected to be a major empirical concern for this study. For example, in a country as small as Ireland, cross-county commuting and migration are common and likely to induce spatial dependence. For this reason, we follow the approach proposed by Mastromarco, Serlenga \& Shin (2016, JAE) who employ the Common Correlated Effects Estimator due to Pesaran (2006, Econometrica) and the Interactive Fixed Effects Estimator due to Bai (2009, Econometrica), which both allow for weak and strong forms of cross-sectional dependence. The estimated unobserved factor structure constitutes a time-varying measure of technical efficiency.
Furthermore, we examine the de-factored residuals with regard to spatial dependence. This follows the framework employed in Holly, Pesaran & Yamagata (2010, J of Econometrics) and Bailey, Holly & Pesaran (2016, JAE) who analyse de-factored residuals for spatial dependence. We make use of recent theoretical advances in high-dimensional statistics to estimate spatial effects, without relying on a pre-specified spatial weights matrix. Specifically, Medeiros & Mendes (2016, J of Econometrics) prove that the Adaptive Lasso is model selection consistent, even if the errors are non-Gaussian, serially dependent and heteroskedastic. Extracting the spatial dependence structure from the de-factored residuals using the Adaptive Lasso allows us to refine the estimates of technical inefficiency, and attribute the difference to spatial effects.
Preliminary results identify the county of Cork as the leader in terms of technical efficiency. We show that, in the case of Ireland, technical efficiency is strongly driven by investment and location decisions of individual multinational companies. Lastly, we discuss the distorting effect of transfer pricing and explore to what extent the results can be explained by the divergence of human capital.
Spatial or cross-sectional dependence is expected to be a major empirical concern for this study. For example, in a country as small as Ireland, cross-county commuting and migration are common and likely to induce spatial dependence. For this reason, we follow the approach proposed by Mastromarco, Serlenga \& Shin (2016, JAE) who employ the Common Correlated Effects Estimator due to Pesaran (2006, Econometrica) and the Interactive Fixed Effects Estimator due to Bai (2009, Econometrica), which both allow for weak and strong forms of cross-sectional dependence. The estimated unobserved factor structure constitutes a time-varying measure of technical efficiency.
Furthermore, we examine the de-factored residuals with regard to spatial dependence. This follows the framework employed in Holly, Pesaran & Yamagata (2010, J of Econometrics) and Bailey, Holly & Pesaran (2016, JAE) who analyse de-factored residuals for spatial dependence. We make use of recent theoretical advances in high-dimensional statistics to estimate spatial effects, without relying on a pre-specified spatial weights matrix. Specifically, Medeiros & Mendes (2016, J of Econometrics) prove that the Adaptive Lasso is model selection consistent, even if the errors are non-Gaussian, serially dependent and heteroskedastic. Extracting the spatial dependence structure from the de-factored residuals using the Adaptive Lasso allows us to refine the estimates of technical inefficiency, and attribute the difference to spatial effects.
Preliminary results identify the county of Cork as the leader in terms of technical efficiency. We show that, in the case of Ireland, technical efficiency is strongly driven by investment and location decisions of individual multinational companies. Lastly, we discuss the distorting effect of transfer pricing and explore to what extent the results can be explained by the divergence of human capital.
Dr. Bettina Becker
Assistant Professor
Aston Business School
The effectiveness of regional, national and EU support for innovation in the UK and Spain
Author(s) - Presenters are indicated with (p)
Bettina Becker (p), Stephen Roper , Jim Love
Abstract
Innovation policy aims to stimulate innovation and hence firm-level productivity and growth. Here, we use data from the national innovation panel surveys in the UK and Spain over the 2004 to 2012 period to explore the effectiveness of regional, national and EU innovation support in promoting the extent of innovation activity, its novelty, and market success. Allowing for potential selection effects, our results suggest that regionalised support is most influential in increasing the probability of undertaking both process and organisational innovations. For both the UK and Spain, national innovation support is associated with a higher probability of product or service innovation, and the degree of novelty of product or service innovations. In terms of innovation success (sales) we see a rather different pattern in the UK and Spain. In the UK only regionalised support is associated with increased innovative sales. In Spain, innovative sales are influenced by both regional, national and EU support measures. Our results therefore suggest that moves towards more centralised innovation policy in the UK since 2012 may have impacted negatively on firms’ ability to effectively commercialise new innovations.
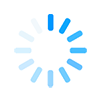