G20-O3 Methods in Regional Science or Urban Economics
Tracks
Ordinary Sessions
Wednesday, August 30, 2017 |
4:00 PM - 5:30 PM |
HC 1315.0037 |
Details
Chair: Yuri Yegorov
Speaker
Dr. Tomasz Kossowski
Assistant Professor
Adam Mickiewicz University
Spatial autocorrelation index for symbolic data - how to measure it?
Author(s) - Presenters are indicated with (p)
Tomasz Kossowski (p), Justyna Wilk
Abstract
Regional studies cover a spectrum of phenomena and problems including social, economic and environmental ones, which refer to spatial units. According to the raising number of spatial surveys and databases, contemporary spatial analyses deal with two problems: very detailed or, in contradiction, imprecise phenomena description. The first one results in data multiplication (e.g. time-series data), while the second one in data generalization (e.g. country-level data).
Both of them are a serious limitation for studies of spatial autocorrelation. One of the frequently used approaches is to determine the “average” values and apply well-known measures of spatial autocorrelation, e.g. Moran’s I statistics. But this operation may affect the studies’ results and deprive them from the relevant interpretation of real spatial processes.
This paper deals with huge and imprecise datasets which is a domain of symbolic data analysis introduced by Bock and Diday (2000), Billard and Diday (2003), Diday and Noirhomme-Fraiture (2008), Brito (2014). This paper introduces a new spatial autocorrelation index which operates on symbolic data, its properties and limitations, and its empirical validation using real spatial data set.
References
Billard L., Diday E., 2003, Symbolic Data Analysis. Conceptual Statistics and Data Mining, Wiley, Chichester.
Bock, H.H., Diday, E. (Eds.), 2000. Analysis of symbolic data. Exploratory methods for extracting statistical information from complex data, Springer-Verlag, Berlin, Heidelberg, pp. 153-165
Brito, P., 2014. Symbolic Data Analysis: another look at the interaction of Data Mining and Statistics, WIREs Data Mining and Knowledge Discovery, 4, 4, 281-295.
Cliff A.D., Ord J.K., 1973, Spatial autocorrelation, Pion, London.
Diday, E., Noirhomme-Fraiture, M. (Eds.), 2008. Symbolic data analysis and the Sodas software, John Wiley & Sons, Chichester.
Moore R., 1966, Interval analysis, Prentice-Hall Inc., Englewood Cliffs N.J.
Both of them are a serious limitation for studies of spatial autocorrelation. One of the frequently used approaches is to determine the “average” values and apply well-known measures of spatial autocorrelation, e.g. Moran’s I statistics. But this operation may affect the studies’ results and deprive them from the relevant interpretation of real spatial processes.
This paper deals with huge and imprecise datasets which is a domain of symbolic data analysis introduced by Bock and Diday (2000), Billard and Diday (2003), Diday and Noirhomme-Fraiture (2008), Brito (2014). This paper introduces a new spatial autocorrelation index which operates on symbolic data, its properties and limitations, and its empirical validation using real spatial data set.
References
Billard L., Diday E., 2003, Symbolic Data Analysis. Conceptual Statistics and Data Mining, Wiley, Chichester.
Bock, H.H., Diday, E. (Eds.), 2000. Analysis of symbolic data. Exploratory methods for extracting statistical information from complex data, Springer-Verlag, Berlin, Heidelberg, pp. 153-165
Brito, P., 2014. Symbolic Data Analysis: another look at the interaction of Data Mining and Statistics, WIREs Data Mining and Knowledge Discovery, 4, 4, 281-295.
Cliff A.D., Ord J.K., 1973, Spatial autocorrelation, Pion, London.
Diday, E., Noirhomme-Fraiture, M. (Eds.), 2008. Symbolic data analysis and the Sodas software, John Wiley & Sons, Chichester.
Moore R., 1966, Interval analysis, Prentice-Hall Inc., Englewood Cliffs N.J.
Dr. Fernando Lopez
Associate Professor
Universidad Politécnica de Cartagena
A Scan test for spatial groupwise heteroscedasticity in cross-sectional models with an application on houses prices in Madrid
Author(s) - Presenters are indicated with (p)
Fernando Lopez (p), Julie Le Gallo, Coro Chasco
Abstract
Abstract: We propose a Scan test for the presence of spatial groupwise heteroskedasticity in cross-sectional data. The Scan approach has been used in different fields before, including spatial econometric models, to detect instability in mean values of variables or regression residuals. In this paper, we extend its use to second order moments. Using large Monte Carlo simulations, we check the reliability of the proposed scan procedure to detect instabilities in the variance, the size and power of the test and its accuracy to find spatial clusters of observations with similar variances. Finally, we illustrate the usefulness of this test to improve the specification search in a spatial hedonic model with an empirical application on housing prices in Madrid.
Ms Karen Alejandra Miranda Gualdrón
Phd Student
Universitat Rovira i Virgili
Dr. Yuri Yegorov
Senior Researcher
Economica
The Role of 2-Dimensional Continuous Space for Models in Regional Science
Author(s) - Presenters are indicated with (p)
Yuri Yegorov (p)
Abstract
Current economic science evolves without substantial use of models of land in continuous space and thus is unable to tackle important problems. While the idea of continuous space was introduced by von Thunen (1826) and later elaborated in 1930s in the works of Hotelling, Losch and Christaller, many new economic models started to treat space discretely. While regional science and urban economics uses continuous space, it dimensionality and topology is not always real. It is important to admit that continuous space models are widely used in urban economics. The core model is about central business district. However, it is mostly used for 1-dimensional case rather than for a radially symmetric city. It will be discussed later why 2-dimensional case might be important for consideration also in the context of urban economics.
Czech (2013) has attracted our attention to several problems. First, he mentioned high role of American economist of the 19th century Henry George who has introduced land as an important production input but was forgotten in later. This resulted in keeping only labor and capital in the production function in macroeconomic growth models. Before it was not so much a problem, but as we have approached the limit of land use (that is in limited supply contrary to capital), this becomes important. Second, he talks about ecological footprints and compares agriculture with the basic trophic level in an ecosystem that should have higher mass. However, current financial accounting highly underestimates the role of agriculture in the produced GDP. Since our use of even renewable resources is already higher than recreating capacity of the Earth, this limit (of land use) will be responsible for the declining economic growth in future.
There are 2 important properties of space: a) land is a measure of an area given by a subset in 2-dimensional space, b) distance between points is Euclidean. If we want to measure transport costs, they are proportional to distance. The minimal distance between any two points in given by Euclidean metrics. Real distance can differ: it can be city block distance in regularly shaped cities, or even more complex since real roads can have curvature. Both the concept of distance and the link between location and land area are missing if we use models with discrete points in space. The paper presents examples where using such space creates new results.
Czech (2013) has attracted our attention to several problems. First, he mentioned high role of American economist of the 19th century Henry George who has introduced land as an important production input but was forgotten in later. This resulted in keeping only labor and capital in the production function in macroeconomic growth models. Before it was not so much a problem, but as we have approached the limit of land use (that is in limited supply contrary to capital), this becomes important. Second, he talks about ecological footprints and compares agriculture with the basic trophic level in an ecosystem that should have higher mass. However, current financial accounting highly underestimates the role of agriculture in the produced GDP. Since our use of even renewable resources is already higher than recreating capacity of the Earth, this limit (of land use) will be responsible for the declining economic growth in future.
There are 2 important properties of space: a) land is a measure of an area given by a subset in 2-dimensional space, b) distance between points is Euclidean. If we want to measure transport costs, they are proportional to distance. The minimal distance between any two points in given by Euclidean metrics. Real distance can differ: it can be city block distance in regularly shaped cities, or even more complex since real roads can have curvature. Both the concept of distance and the link between location and land area are missing if we use models with discrete points in space. The paper presents examples where using such space creates new results.
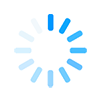